What we’re all getting wrong about ADHD
发布时间 2023-08-06 23:00:00 来源
摘要
We’ve all heard of ADHD, or Attention Deficit Hyperactivity Disorder. But there’s actually a lot scientists don’t for sure know about the condition. From its causes, to what actually defines the disorder – or if it’s a disorder at all – is all hotly debated.
To guide us through the latest ADHD research, we’re joined by one of the world’s biggest experts on the topic, Professor Edmund Sonuga-Barke. He’s professor of Developmental Psychology, Psychiatry and Neuroscience at King’s College London.
Learn more about your ad choices. Visit podcastchoices.com/adchoices
GPT-4正在为你翻译摘要中......
中英文字稿 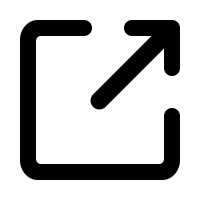
Thank you so much to friend Whiting me. It's such a pleasure to be talking about these things here in my own department. And it's so cool to see how many interesting things are happening right here. So I'm going to talk about keeping AI under control with mechanistic interpretability. And in particular, how I think we physicists have a great opportunity to help with this.
非常感谢朋友们对我的支持。我非常高兴能够在自己的部门里讨论这些事情。看到这里发生了许多有趣的事情真是太棒了。所以我打算谈一谈如何通过机械的可解释性来控制人工智能,并且我认为我们物理学家在这方面有巨大的机会可以提供帮助。
So first of all, why might we want to keep AI under control? Well, obviously, as we've heard this morning, because it's getting more and more powerful, we've all seen this paper from Microsoft arguing that the GPT-4 is already showing sparks of artificial general intelligence. Here is Yoshua Benjio. Now I'll reach the point where there are AI systems like between humans meaning they can pass the trade test. So I can debate whether or not GPT-4 passes a Turing test. But Yoshua Benjio should certainly get a vote in that debate, since he's one of the Turing Award winners, the equivalent of the Nobel Prize for AI. And this growth in progress is obviously also, as you know, started freaking a lot of people out.
首先,为什么我们要控制人工智能呢?嗯,显然,正如我们今天早上听到的那样,因为它越来越强大,我们都看到了微软的这篇论文,声称GPT-4已经展现出人工通用智能的迹象。这是Yoshua Benjio。现在我要说的是,现在已经有了像人类之间的人工智能系统,也就是说它们可以通过图灵测试。所以我可以讨论GPT-4是否通过了图灵测试。但是Yoshua Benjio在这场辩论中肯定应该有发言权,因为他是图灵奖得主之一,相当于人工智能界的诺贝尔奖。而且正如你们知道的,这种进步的增长显然也让很多人感到恐惧。
Here we have his Turing Award co-winner Jeff Hinton. I'm not sure if the audio is actually going out. Is it? Are we close to the computers coming up with their own ideas for improving the sound? Yeah, two months. And then it could just go. That's an issue, right. We have to think hard about how to control that. Yeah, can we? We don't know. We haven't been there yet, but we can try. OK, let's see. It was kind of concerning. Yes. And then piling on some opman, CEO of OpenAI, and then of course it has given us chat GPT-4, GPT-4. Have this to say? And the bad case, and I think this is important to say, is like lights out for all of us. Lights out for all of us. Doesn't sound so great. And of course, then we had a bunch of us who called for a pause and an open letter. And then we had shortly after that, this bunch of AI researchers talking about how this poses a risk of extinction, which is all over the news. Specifically, it was the shortest open letter I've ever read. And I just want sentence mitigating the risk of extinction from AI should be a global priority alongside other societal scale risks, such as pandemics and nuclear war. So basically, the whole point of this was that it mainstreamed the idea that, hey, maybe we could get wiped out. So we really should keep it under control. And the most interesting thing here, I think, is who signed it? You have not only top academic researchers who don't have a financial conflict of interest, people like Jeff Hinton, Yoshua Benjo. But you also have the CEOs here, Demis Asab, is from Google Deep Mind, Sam Altman again, Dari Amade, et cetera. So a lot of reasons why we should keep AI under control.
在这里,我们有图灵奖得主杰夫·辛顿。我不确定声音是否正在输出。是吗?计算机是否接近提出改善声音的自己的想法?对,还有两个月。然后它就能够开始了。这是个问题,对吧。我们必须认真考虑如何控制它。是的,我们可以吗?我们不知道。我们还没有到那个阶段,但我们可以尝试。好吧,让我们看看。这有点让人担心。是的。然后是OpenAI的首席执行官Openman,当然它已经给我们带来了Chat GPT-4,GPT-4。有什么要说的吗?还有最糟糕的情况,我认为这一点很重要,就是对我们所有人来说都是灾难。对我们所有人来说都是灾难。听起来不那么好。当然,然后我们有一些人联名呼吁暂停并发表了一封公开信。然后我们不久之后,这群人工智能研究人员开始讨论这就是濒临灭绝的风险,这一消息遍及新闻界。特别是,这是我读过的最简短的公开信。我只想说句话,减轻人工智能造成的灭绝风险应当成为与大规模社会风险(如瘟疫和核战争)并列的全球优先事项。因此,这次事件的核心便是,它使得这个想法成为主流观点,即“也许我们会被消灭”,所以我们真的应该将其控制起来。而这里最有趣的事情,我认为,是谁签署了这封信?不仅仅是一些没有经济利益冲突的顶级学术研究人员,像杰夫·辛顿、Yoshua Benjo这样的人,还有一些首席执行官,比如谷歌Deep Mind的Demis Asab,再比如Sam Altman,Dari Amade等等。所以我们有很多理由应该将人工智能控制起来。
How can we help? I feel that, first of all, we obviously should. And Peter, earlier this morning, gave a really great example of how I think we really can help by opening up the black box and getting to a place where we're not just using ever more powerful systems that we don't understand, but where we're instead able to understand them better. This has always been the tradition in physics when we work with powerful things. If you want to get a rocket to the moon, you don't just treat it as a black box and you fired. Oh, that one went a little too far to the left. Let's aim a little farther to the right next time. No, what you do is you figure out the laws of, you figure out Einstein's laws of gravitation, you figure out Dari Amade, et cetera. And then you can be much more confident that you're going to control what you build.
我们可以如何帮助?我觉得首先,显然我们应该帮助。而且,早上彼得给了一个非常好的例子,我觉得我们可以通过打开黑匣子并进入一个更好地理解它们的状态,从而真正地提供帮助。当我们处理强大的东西时,物理学始终秉持这一传统。如果你想把火箭送上月球,你不会简单地把它当作一个黑匣子然后点火。哦,那个飞得有点太左了,下次我们稍微往右边打一点。不,你需要找出引力的定律,找出爱因斯坦的引力定律,找出达里阿玛的定律等等。然后你才能更有信心地控制自己所建造的东西。
So this is actually a field which has gained a lot of momentum quite recently. It's a very small field still. It's known by the nerdy name of mechanistic interpretability to give you an idea of how small it is.
所以最近这个领域实际上获得了很大的动力。它还是一个非常小的领域。它被称为“机械性可解释性”以给你一个了解它有多小的概念。
If you compare it with neuroscience, and you think of this as artificial neuroscience, neuroscience is a huge field, of course. And look how few people there are here at MIT at this conference and I organized just two months ago. This was the biggest conference by far in this little nascent field, OK? So that's the bad news. It's very few people working on it.
如果你将其与神经科学进行比较,并将其视为人工神经科学,那神经科学是一个庞大的领域,当然了。看看这个麻省理工学院的会议上有多少人,而我仅仅在两个月前组织了这个会议。这是迄今为止在这个新生领域中最大的一次会议,明白吗?所以这是个不好的消息。研究这个领域的人非常少。
But the good news is, even though there are so few, there's already been a lot of progress, remarkable progress. I would say more progress in this field than in all of big neuroscience in the last year. Why is that?
但好消息是,尽管数量很少,但已经取得了很多进展,非常显著的进展。我会说,在过去一年中,在这个领域取得的进展比整个大脑神经科学领域的进展还要多。为什么会这样呢?
It's because here you have a huge advantage over ordinary neuroscience in that, first of all, to study the brain with 10 to the 11 neurons. You have a hard time reading out more than 1,000 at a time. You need to get IRB approval for all sorts of ethics reasons and so on. Here, you can read out every single neuron all the time. You can also get all the synaptic weights. You don't even have to go to the IRB either. And you can use all these traditional techniques where you actually mess with the system and see what happens that we love to do in physics.
这是因为在这里,你对普通神经科学有一个巨大的优势,首先,你需要研究具有10的11次方个神经元的大脑。但你一次最多只能读出1000个神经元。你需要获得各种伦理原因的IRB批准等等。而在这里,你可以随时读出每一个神经元。你还可以获取所有的突触权重,甚至不需要去申请IRB批准。而且你可以使用所有这些我们喜欢在物理学中做的对系统进行实际干预并观察结果的传统技术。
And I think there are three levels of ambition that can motivate you to want to work on mechanistic interpretability, which is, of course, what I'm trying to do here to encourage you to work more on this.
我认为有三个层次的雄心壮志可以激励你想要在机械解释性方面进行工作,当然,这也是我在这里试图做的,鼓励你更多地投入到这方面的工作中。
The goal of it is the first lowest ambition level is just when you train a black box neural network on some data to do some cool stuff to understand well enough that you can diagnose its trustworthiness, make some assessment of how much you should trust it. That's already useful.
它的目标是通过在某些数据上训练黑盒神经网络实现一些酷炫的功能,并对其进行足够的理解,以便能够诊断其可靠性,评估应该相信它的程度。这已经是很有用的了。
Second level of ambition, if you take it up a notch, is to understand it so well that you can improve its trustworthiness.
如果你再进一步,第二层雄心壮志就是要彻底理解它,从而能提升它的可信度。
And the ultimate level of ambition, and we are very ambitious here at MIT, is to understand it so well that you can guarantee trustworthiness. We have a lot of work at MIT on formal verification where you do mathematical proofs that a code is going to do what you want it to do.
在麻省理工学院,我们非常雄心勃勃,而最终的目标是彻底理解代码,以至于你可以保证其可信性。在麻省理工学院,我们进行了大量关于形式验证的工作,其中通过数学证明一个代码将会按照你的意愿运行。
Proof carrying code is a fat popular topic in computer security where the it's a little bit like if I was check your in reverse, if I was check your refused to run your code, if it can prove that it's harmful. Here, instead, the operating system says to the code, give me a proof that you're going to do what you say you're going to do. And if the code can't present the proof that the operating system can check it, it won't run it.
自证代码是计算机安全领域的一个热门话题,有点类似于倒过来检查你的代码。如果它能证明自己是有害的,那么如果我检查你的代码,你会拒绝运行它。在这里,相反地,操作系统对代码说,给我一个证据证明你将按照你所说的去做。如果代码不能提供操作系统可以检查的证据,它将不会运行。
It's hopeless to come up with rigorous proofs for neural networks because it's like trying to prove things about spaghetti. But the vision here is if you can use AI to actually mechanistically extract out the knowledge that's been learned, you can re-implement it in some other kind of architecture, which isn't a neural network, which really lends itself to formal verification.
很难为神经网络提供严格的证明,就像试图证明关于意面的事情一样。但目前的展望是,如果能够使用人工智能来机械地提取已经学习到的知识,就可以在其他种类的架构中重新实现它,而这种架构并不是神经网络,这将非常适合进行形式化验证。
If we can pull off this moonshot, then we can trust systems much more intelligent than us because no matter how smart they are, they can't do the impossible.
如果我们能完成这个激进目标,那么我们就能够相信比我们更智能的系统,因为无论它们有多聪明,它们也无法做到不可能的事情。
So in my group, we've been having a lot of fun working on extracting learned knowledge from the black box in the mechanistic interpretability spirit. You heard, for example, my grad student, Michelle talked about this quantum thing recently. And I think this is an example of something which is very encouraging because if this quantum hypothesis is true, you can do with divide and conquer.
在我们的小组里,我们一直在以机械解释的精神中从黑匣子中提取学到的知识,这样做非常有趣。你可能听过我研究生米歇尔最近谈到了量子理论。我认为这是一个非常令人鼓舞的例子,因为如果这个量子假设是真的,你可以采用分而治之的方法解决问题。
You don't have to understand the whole neural network all at once. But you can look at the street, quantitative learning, study them separately, much like we physicists don't try to understand the status center all at once. First, we try to understand the individual atoms that it's made of, and then we can work our way up to solid state physics and so on.
你不必一次性完全了解整个神经网络。但你可以从街道,定量学习等方面来观察并分别研究它们,就像物理学家不会试图一次性理解整个状态中心一样。首先,我们尝试理解构成其的单个原子,然后可以逐步了解固体物理学等更高层次的知识。
Also reminds me a little bit of Minsky's Society of Mines where you have many different systems working together to provide very powerful things.
这也让我有点想起明斯基的“矿山社会”,在那里,许多不同的系统共同运作,提供了非常强大的事物。
I'm not going to try to give a full summary of all the cool stuff that went down at this conference, but I can share, there's a website where we have all the talks on YouTube if anyone wants to watch them later.
我不打算尝试对这次会议中发生的所有很酷的事情做全面总结,但是我可以分享一个网站,我们把所有的演讲都放在YouTube上,如果有人想以后观看的话,可以去那个网站看。
But I want to just give you a little more nerd flavor of how tools that many of you here know, as physicists, are very relevant to this. Things like phase transitions, for example. So we already heard a beautiful talk by Jacob Andreas about knowledge representations.
但是我想给大家更多以物理学家身份认知的方式向你们介绍一些工具,这些工具对于你们许多人来说非常相关,比如相变等等。所以你们已经听到Jacob Andreas关于知识表示的精彩演讲了。
There's been a lot of progress on figuring out how large language models represent knowledge, how they know that the Eiffel Tower is in Paris and how you can change the weights so that it thinks it's in Rome, et cetera, et cetera.
关于大型语言模型如何表示知识、如何知道埃菲尔铁塔在巴黎,以及如何调整权重使其认为它在罗马等问题,取得了许多进展,等等。
We did a study on algorithmic data sets where we found phase transitions. So if you try to make the machine learning learn a giant multiplication table, this could be for some arbitrary group operation. So something more interesting than standard multiplication. Then if there's any sort of structure here, if this operation is, for example, commutative, then you only really need the training data for about half of the entries and you can figure out the other half because it's a symmetric matrix. If it's also associative, then you need even less, et cetera. So as soon as the machine learning discovers some sort of structure, it might learn to generalize.
我们对算法数据集进行了一项研究,发现了相变现象。因此,如果你尝试让机器学习学习一个巨大的乘法表,这可能是某个任意的群操作。所以比标准乘法更有趣的东西。然后,如果这里有任何形式的结构存在,例如,如果这个操作是可交换的,那么你只需要训练数据的一半,因为它是对称矩阵,你可以推断出另一半。如果它还是满足结合律,那么你需要的数据量甚至更少,依此类推。所以一旦机器学习发现了某种结构,它就可能学会进行泛化。
So here's a simple example. Edition, module 59. We train an inner learner to do this. We don't give it an input as numbers. We just give it each of the numbers from zero to 58 as a symbol. So it doesn't have any idea that they should be thought of as numbers and it represents them by embedding them in some internal space. And then we find that exactly at the moment when it learns to generalize, to unseen examples, there's a phase transition in how it represents in the internal space. We find that it was in a high dimensional space, but everything collapses through two dimensional hyperplane. I'm showing you here in a circle. Boom. That's of course exactly like the way we do edition, module 12 when we look at a clock, right? So it finds a representation where it's actually adding up angles which automatically captures in this case the commutativity and associativity. And I suspect this might be a general thing that happens in learning language and other things also that it comes up with a very clever representation that is such that it geometrically encompasses a lot of the key properties that let's it generalize.
所以这里有一个简单的例子。第59版,模块化。我们训练一个内部学习器来做这个。我们没有将输入作为数字给它。我们只是将零到58之间的每个数字作为符号给它。所以它并不知道它们应该被视为数字,并且通过将它们嵌入到某个内部空间中来表示它们。然后我们发现,当它学会推广到看不见的例子时,它在内部空间中的表示发生了相变。我们发现它原本在一个高维空间中,但是一切都坍缩成了一个二维超平面。在这里,我用一个圆形来展示。嘭。当然,这当然就像我们在看时钟时执行模12加法的方式,对吧?所以它找到了一种表示,在这种表示中,它实际上将角度相加,这自动捕捉到了交换性和结合性。我怀疑这可能是在学习语言和其他事物中发生的一般现象,它会提出一个非常巧妙的表示,从而在几何上包含了许多让它能够推广的关键属性。
We do a lot of phase transition experiments also. We tweak various properties of the neural network and see that sometimes it, so there's one region of, if you think of this being, you know, water, you could have pressure and temperature on your phase diagram, but here there are various other nerdy machine learning parameters and there's some, you get these phase transition boundaries between where it just learns properly, where it can generalize, where it fails to generalize and it never learns anything or where it just over fits this is for the example of just doing regular addition. So you see it learns to put the symbols on a line rather than a circle in the cases where it works out.
我们也做了很多相变实验。我们调整神经网络的各种属性,有时候会看到一些区域,在这个区域,你可以将其想象成水的相图上的压力和温度,但是在这里,存在着各种令人困惑的机器学习参数,而且有些地方存在相变的边界,其中它能够正确学习,能够泛化,不能泛化而无法学到任何东西,或者只是过拟合。举个例子,我们只是做了普通的加法,在那些它能够学习的情况下,你会看到它学会将符号排列成一条线,而不是一个圆圈。
So I wanna leave a little bit of time for questions, but the bottom line I would like you to take away from all this is I think it's too pessimistic to say, oh, you know, we're forever just gonna be stuck with these black boxes that we can never understand. Of course, if we convince ourselves that it's impossible, where we're gonna fail, that's the best recipe for failure. I think it's quite possible that we really can understand enough about very powerful AI systems that we can have very powerful AI systems that are provably safe and physicists can really help a lot because we have a much higher bar before we mean by understanding things than a lot of our colleagues in other fields. And we also have a lot of really great tools. We love studying nonlinear dynamical systems. We love studying phase transitions and so many other things which are turning out to be key in doing this kind of progress.
所以我想给一点时间回答问题,但我希望你能从中明白一点,我认为说我们将永远被困在这些我们永远无法理解的黑盒子里太悲观了。当然,如果我们让自己相信这是不可能的,我们就注定会失败,这是失败的最佳配方。我认为我们完全有可能理解非常强大的人工智能系统的足够多,以至于我们能够拥有可以证明安全的非常强大的人工智能系统。物理学家可以提供很大的帮助,因为我们对于理解事物的标准要求比其他领域的同行更高。而且我们还拥有很多非常出色的工具。我们喜欢研究非线性动力学系统,喜欢研究相变和其他许多有助于取得进展的领域。
So if anyone is interested in collaborating or learning more about mechanistic interpretability and basically studying the learning and execution of neural networks as just yet another cool physical system to try to understand just to read that to me. And let's talk. Thank you. Thank you very much. Does anyone have questions?
如果有人对合作或深入了解具体研究机制可解释性以及将神经网络的学习和执行作为另一个有趣的物理系统来理解感兴趣的话,请给我留言,我们可以交流一下。谢谢,非常感谢。有人有问题吗?
I actually have one to start with. So just sort of you explaining in these last few slides, a lot of the theme sort of seem to be applying like the laws of thermodynamics and other physical laws to these systems. And the parallel I thought of is the field of biophysics also sort of emerged out of this, right? Applying physical laws to systems that were considered too complex to understand before we really thought about it carefully. Is there any sort of emerging field like that in the area of AI or understanding neural networks other than that little conference you just mentioned? Or is that really all that's there right now?
我实际上有一个开始的问题。所以你在最后几张幻灯片中的解释,许多主题似乎都是将热力学法则和其他物理法则应用到这些系统中。我想到的类似情况是生物物理学领域也是从这样开始的,对之前被认为过于复杂而无法理解的系统应用物理法则。在AI领域或者理解神经网络方面,是否有类似兴起的领域,除了你刚提到的那个小型会议之外?还是目前只有那么一点内容?
There's so much room for there to really be an emerging field like this. And I invite all of you to help build it. It's obviously a field which is not only very much needed but it's just so interesting.
这个领域有很大的发展空间。我邀请大家一起来推动它的建设。显然,这不仅是非常需要的领域,而且非常有趣。
There have been so many times in recent months when I read a new paper by someone else about this and I'm like, oh, this is so beautiful. You know, another way to think about this is I always tell my students that when they pick tasks to work on, they should look for areas where there's more data where experiment is ahead of theory. That's the best place to do theoretical work. And that's exactly what we have here, right?
最近几个月里,有很多次我读到别人写的新论文时,会感觉"哇,这篇论文太棒了"。你知道,另一种思考方式是,我经常告诉我的学生们,在选择研究任务时,应该寻找实验领先理论的领域,那是进行理论研究的最佳场所。而我们现在正好就是这种情况,对吧?
If you train some system like GPT-4 to do super interesting things or use LAMA-2 that just came out where you have all the parameters, it's an incredibly interesting system. You can get massive amounts of data and we have the most fundamental things we don't understand. It's just like when the LHC turns on or when you first launched the Hubble Space Telescope or the WMAP satellite or something like that, you have the massive amount of data, really cool basic questions. It's the most fun domain to do physics in and yeah, let's build a field around it. Thank you, we've got a question up there.
如果你训练一些像GPT-4这样的系统来做超级有趣的事情,或者使用刚刚发布的LAMA-2,你会拥有所有参数,这是一个非常有趣的系统。你可以获得大量的数据,而我们对其中最基本的事物仍然不理解。就像LHC启动时,或者你第一次发射哈勃太空望远镜或WMAP卫星那样,你会有大量的数据,非常酷的基本问题。这是最有趣的物理领域,是的,让我们围绕它建立一个领域。谢谢,我们有一个问题在那里。
Hi, Professor Tegmark. I was wondering, so most first amazing talk, I love the concept, but I was wondering if it is possible that this approach might not oversee but miss situations in which the language model actually performs very well, not in a sort of like a concise region, like a phase region on parameter space, but rather like in small blobs all around because in most physical systems, we have a lot of parameters that we will have phases and the phases are mostly concise in regions of n dimensions or whatever, and then there are phase transitions, which is the concept here, but it is also like since this is not necessarily a physical system, maybe there might be a situation in which the best way that it performs this in specific combinations of parameters, there are like points or little blobs around, I don't know if my question went through.
你好,Tegmark教授。我一直在思考,您的第一场精彩演讲真是让我着迷,我喜欢这个概念,但我想知道是否有可能这种方法可能不只是忽视,而是错过了一些语言模型表现得非常出色的情况,不仅仅是在某个紧凑区域内,比如参数空间中的某个相位区域,而是在周围的一些小的区域中,因为在大多数物理系统中,我们有很多参数,我们会有相位区域,而这些相位区域大多是在n维空间中的某些区域内,然后还有相变的概念,这就是这里的概念,但由于它不一定是一个物理系统,也许可能存在一种情况,即它在特定的参数组合下表现最佳,有一些点或小的区域周围,我不知道我的问题是否传达清楚。
Yeah, good question. I think I need to explain better. I think my proposal is actually more radical than maybe it's, I could properly explain. I think we should never put something we don't understand, like GPT-4 in charge of the MIT nuclear reactor or any high stakes systems. I think we should use these black box systems to discover amazing knowledge and discover patterns in data, and then we should extract, not stop there and just connect it to the nuclear weapons system or whatever, but we should instead develop other AI techniques to extract out the knowledge that they've learned and be implement them in something else, right?
是的,好问题。我认为我需要更好地解释一下。我认为我的提议实际上比我可能能够适当解释的要激进得多。我认为我们永远不应该让我们不理解的东西,比如GPT-4,掌管麻省理工学院的核反应堆或任何高风险系统。我认为我们应该使用这些黑匣子系统来发现惊人的知识和数据中的模式,然后我们应该提取出来,而不只是停留在那里然后将它连接到核武器系统或其他东西,我们应该研发其他的人工智能技术来提取出它们所学到的知识,并将其应用于其他事物,对吧?
So, to take your physics metaphor again, that's a Galileo when he was four years old, if his daddy threw him a ball, he'd catch it. Because his black box neural network had gotten really good at predicting the trajectory. Then he got older and he's like, wait a minute, these trajectories always have the same shape, but it's a parabola, y equals x squared and so on. And when we send the rocket to the moon, right, we don't put a human there to make poorly understood decisions. We actually have extracted out the knowledge and written the Python code or something else that we can verify.
所以,再用你的物理学隐喻来说,那就是当伽利略四岁的时候,如果他爸爸给他扔个球,他能接住。因为他的黑盒神经网络在预测弹道方面已经变得非常优秀了。后来他长大了,他疑惑了,说等一下,弹道总是有着相同的形状,而且是一个抛物线,就是y等于x的平方之类的。当我们将火箭送往月球时,我们不会让一个对决策知之甚少的人去控制。我们实际上已经将知识提取出来,编写了Python代码或其他可以进行验证的代码。
I think the real power, I think we need to let go of a stop putting an equal sign between large language models and AI. We've had radically different ideas of what AI should be. First, we thought about it in the von Wehrmann paradigm of computation. Now we're thinking about LLMs. We can think of other ones in the future. What's really amazing about neural networks, in my opinion, is not their ability to execute a computation at runtime. They're just another massively parallel computational system and there are plenty of other ones too that are easier to formally verify. What they really shine is in their ability to discover patterns and data to learn. And let's continue using them for that.
我认为真正的力量,我们需要放下一种认为大型语言模型和人工智能是相等的想法。我们对人工智能的理念已经发生了根本性的变化。起初,我们在冯·诺依曼的计算范式中思考它。现在我们在思考LLMs。未来我们也可以思考其他的。在我看来,神经网络真正令人惊奇的不是它们在运行时执行计算的能力。它们只是另一种大规模并行计算系统,还有许多其他更容易进行正式验证的系统。它们真正璀璨的地方在于它们发现模式和学习数据的能力。让我们继续利用它们做这件事。
You could even imagine an incredibly powerful AI that is just allowed to learn but is not allowed to act back on the world in any way. And then you use other systems to extract out what it's learned and you implement that knowledge into some system that you can provably trust. This to me is the path forward that's really safe. And maybe there will still be some kind of stuff which is so complicated we can't prove that it's going to do what we want. So let's not use those things until we can prove them because I'm confident that the set of stuff that can be made provably safe is vastly more powerful and useful and inspiring than anything we have now. So why should we risk losing control when we can do so much more first and provably safely?
你甚至可以想象一个极其强大的人工智能,它只被允许学习,但不能以任何方式对世界产生影响。然后,你可以使用其他系统提取它所学到的东西,并将这些知识应用到一些可以被证明可信的系统中。对我来说,这是一条非常安全的前进路径。也许还会有一些非常复杂的东西,我们无法证明它们会按照我们的意愿行动。所以在我们能够证明之前,我们不应该使用那些东西,因为我相信那些可以被证明安全的事物的集合比我们现在拥有的任何东西都更强大、更有用、更具启发性。所以为什么我们要冒着失去控制的风险,而不是先做更多的事情,来证明它们是安全的呢?
I'll keep my question short.
我会简明扼要地提出我的问题。
So for your first question, is it just an empirical observation or do you have a theoretical model like you do in physics? Right now it's mainly theoretical observation. And actually we have seen many examples of face transitions cropping up in machine learning. And so have many other authors.
对于你的第一个问题,这只是一种凭经验得出的观察,还是像物理学中那样有一个理论模型?目前主要是基于理论观察。实际上,我们已经看到了许多机器学习中涌现出的面部变化的例子。许多其他作者也是如此。
I am so confident that there is a lot of things that are happening and so have many other authors. There is the beautiful theory out there to be discovered. Sort of unified theory of face transitions in learning. And maybe you're going to be the one to first formulate it. I don't think it's a coincidence that these things keep happening like this.
我非常有信心,很多事情正在发生,许多其他作者也是如此。有一种美丽的理论等待我们去发现,那就是面部转变在学习中所形成的统一理论。或许你会成为第一个提出这个理论的人。我不认为这些事情一直以这种方式发生是巧合的。
But this is this gives you all an example of how basic physics like questions there are out there that are still unanswered. Where we have massive amounts of data is clues to guide us towards them. Thank you.
但这也为大家提供了一个例子,即基础物理学中还有许多问题尚未得到解答。我们拥有大量的数据作为线索来引导我们寻找答案。谢谢。
And I think there's probably even in discover, we will probably discover some point in the future even a very deep, unified relationship or duality between thermodynamics and learning dynamics is the hunch I have. Thank you.
我认为在未来的发现中,我们可能会发现热力学和学习动力学之间存在着一种非常深入、统一的关系或二元性。这是我预感到的。谢谢。