AI at Datadog: Monitoring machines in the age of LLMs | Olivier Pomel, CEO of Datadog
发布时间 2024-09-27 11:58:53 来源
摘要
In this episode, we dive deep into the story of how Datadog evolved from a single product to a multi-billion dollar observability ...
GPT-4正在为你翻译摘要中......
中英文字稿 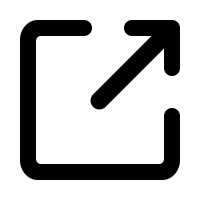
Hi, I'm Matt Turk. Welcome to the Matt podcast. Today, we have a very special episode with Olivier Pomel, the CEO of Datadog. Datadog is a monster of a company and also a great entrepreneurial story, the result of an unlikely journey as it was founded by two French immigrants in 2010 in New York City. At the time when starting an enterprise software company in New York, we're still very much considered a strange idea, this team to fail. Fast forward to today, Datadog has become one of the most exciting tech companies in public markets, who's very impressive stats, almost 29,000 customers around the world, 2.39 billion in revenue, and a 39 billion market cap at the time of recording.
嗨,我是马特·特克。欢迎收听马特播客。今天我们有一集特别节目,嘉宾是Datadog的首席执行官Olivier Pomel。Datadog是一家非常成功的公司,也是一个很棒的创业故事。它由两位法国移民于2010年在纽约市创立,当时在纽约创办一家企业软件公司仍被认为是一个奇怪的想法,不太可能成功。然而,时间快进到今天,Datadog已经成为公共市场上最令人振奋的科技公司之一,其数据令人印象深刻:全球拥有将近29,000家客户,营收为23.9亿美元,市值为390亿美元(录制时的数据)。
In this conversation, we went deep with Olivier and talked about their product strategy. We don't do it like Apple, we don't disappear in a basement for three years and then ship a fully formed product that takes the world by storm. Instead, from the earliest days, we work with design partners, we work with customers, and we try to ship something to them as quickly as possible. And have they been able to add product after product to the platform over the years? Scaling a successful product and starting a new product are very different motions. People feel very differently about it. Why Datadog was initially careful about machine learning and AI, but this system's tend to be more force-negative than force-positive.
在这次谈话中,我们与Olivier进行了深入交流,讨论了他们的产品策略。我们不像苹果那样,在地下室消失三年,然后推出一个令世界震惊的完整产品。相反,从一开始,我们就与设计合作伙伴和客户合作,并尽量尽快向他们交付产品。那么,他们是否能够在这些年中不断为平台增加新产品?扩展一个成功的产品和启动一个新产品是非常不同的行动,人们对此有着不同的感受。为什么最初Datadog对于机器学习和人工智能持谨慎态度,因为这些系统往往倾向于产生负面影响而非正面效应。
Basically, this system is not sure, it's probably not going to tell you anything, because if it tells you things are going wrong twice and you don't believe it, you're never going to look at it again. And their current AI strategy as they double down products like watchdog, bits AI, and the new Foundation model, Toto. Olivier is one of the most thoughtful founders out there, so please enjoy this terrific conversation with him.
基本上,这个系统并不确定,它可能不会告诉你任何事情。因为如果它告诉你两次说事情出错了,而你不相信它,你就不会再去看它了。他们目前的AI策略是加倍投入像Watchdog、Bits AI和新的基础模型Toto这样的产品。Olivier是最有想法的创始人之一,所以请欣赏这段与他的精彩对话。
Welcome, Olivier. Or should I say, Janv new, like I hope that people are ready for the sound of two French peoples with each other in English. Yes, the problem, usually when that happens, like the accent devolves into something that's very, very French. We will try not to get there. People have been warned. You've been in New York for 25 years, right? Or something you were writing in the late 90s?
欢迎,Olivier。或者我应该说“Janv new”,就像我希望大家准备好听到两个法国人用英语交谈一样。是的,通常当这种情况发生时,口音会变得非常非常法国化。我们会尽量避免这种情况。已经提醒过大家了。 你在纽约已经待了25年,对吗?或者你在90年代末就开始写作了?
Yeah, I moved here in 1999. Okay. Yeah, same general thing. Very comparable journey is obviously other than the fact that you build a $39 billion market cap company and I'm a VC with a podcast, but other than that, pretty close. What did you stay in New York? Well, first because it was fun. So I moved in 1999, I thought I was 10, six months. I had an internship at IBM in research, which was a very interesting place at the time. And then it was a tail end of the dot-com boom. So there was a lot going on at that time in New York. There was not much going on in France from a tech perspective.
好的,我在1999年搬到这里。哦,和你的经历基本上差不多,当然除了你建立了市值390亿美元的公司,而我是一名拥有播客的风投。但除此之外,还挺接近的。你为什么留在纽约呢?首先,因为这里很有趣。我在1999年搬过来,本以为只待十个月或半年。我在IBM研究部门有一个实习机会,那时候是个非常有趣的地方。那时正好是互联网泡沫的尾声,纽约有很多事情发生。从科技发展的角度来看,法国当时没什么动静。
So I thought it was a great time to start to stay and join startups. It was interesting. Of course, then there was dot-com crash, 9-11, all of that stuff. So that was less fun. But by that time, I was very attached to Siri. I liked the dynamism. I liked the cultural aspects of the Siri and it's decided to stay. And I stayed until it was until 2010 that I started that adog in New York. And what do you make of the current or recent explosion of the French tech ecosystem? Because I do remember saying the exact story. Like I came here and then there was basically not much to go back for in France and tech at the time.
所以我认为那是一个很好的时机去加入创业公司。当时的确很有意思。当然,后来发生了互联网泡沫破裂、911事件之类的事情,那段时间就不太好玩了。但那时候我已经对Siri产生了很深的感情。我喜欢Siri的活力和文化,所以决定留下来。我一直待到2010年,那时我在纽约开始了另一段旅程。对于最近法国科技生态系统的爆发,你怎么看?因为我记得有过类似的经历:我来到这里时,感觉当时法国的科技领域并没有太多可发展的空间。
Yeah, I think it's amazing. I think it when I left, there was not much going on. When I started that adog also in 2010, there was also not much going on. It was difficult to start companies. Few people wanted to work in startups in France. It was difficult to get funding and all of that stuff. And I think now it's changed a lot. Like there are many companies that are getting started, that are very dynamic, doing very well. I think we still have to see them scale to large companies in France, in Europe more broadly. It hasn't happened yet. So the ecosystem is still young and I would stay still fragile from that perspective. Especially, I mean, of course, compared to the Bay Area, but also compared to the New York ecosystem.
是的,我认为这太棒了。我觉得在我离开的时候,情况并不太好。我在2010年开始创办公司时,情况也并没有好转。在法国创业很困难,很少有人愿意在创业公司工作,融资也很难。而我觉得现在已经有很大变化了。有很多公司正在成立,并且非常有活力、发展得很好。但我认为我们仍需观望它们能否成长为法国甚至整个欧洲的大型公司。目前还没有这样的例子。因为在这方面,整个生态系统依然年轻,也可以说仍然脆弱。尤其是,与湾区相比,当然也包括与纽约的生态系统相比。
Yeah, and exit, right? Scale and exit. Yeah, exactly. Because France hasn't had that cycle of people making money and redistributing it into the ecosystem. Yeah, it has been, but typically earlier, like smaller exits. And I think they need to have more of the later ones. Cool. So maybe for context, so you and I did something sort of similar a few years ago in the context of data driven NYC, which is the monthly data I made up I've been running for 11 years now.
好的,翻译成中文如下:
是的,然后退出,对吧?扩张和退出。是的,确实如此。因为法国还没有经历过这样的周期:人们赚到钱然后再把它重新投入到生态系统中。以前是有的,但通常是更早期、规模较小的退出。我觉得他们需要有更多晚期的退出项目。好的,为了提供背景信息,你和我几年前在数据驱动的纽约进行过类似的事情,那是我经营了11年的一个月度数据活动。
And in some ways, this podcast, the Mad Podcast, is a spinoff from it. But that was a really good conversation that stood the test of time as I was prepping for this. I listened to it again. And I would encourage people to go back to it, to hear sort of the basics, like the data doc story, the founding story, the initial vision, where the name comes from, all the things. And as real YouTubers say, we will put that in the show notes. So people should check that out.
在某些方面,这个播客——疯狂播客(Mad Podcast)是它的一个衍生节目。不过,那次谈话非常精彩,经得起时间的考验,当我做准备时又重听了一遍。我建议大家回去听一下,可以了解一些基本的内容,比如数据文档的故事、创始故事、最初的愿景和名称的由来等等。作为真正的YouTuber所说的,我们会把这些放在节目备注里,所以大家应该查看一下。
But for today, we're going to try and do something a little different, more focused on product, more focused on all things data and AI at Data Doc. Maybe a great place to start will be the usual 101 on Data Dog, your elevator pitch that you've probably given about 10 million times at this point. Yeah, so we're Data Dog. We do observability and security. We do it for cloud environments. So we sell to engineers, basically, that run applications and infrastructure in cloud environments. And we do it for companies that are big and small.
但是今天,我们要尝试做一些有点不同的事情,更专注于产品,更专注于Data Doc的所有数据和人工智能相关的内容。也许一个很好的开始会是Data Dog的101讲解,你可能已经做过大约1000万次的电梯演讲。 是的,我们是Data Dog。我们提供可观察性和安全性服务,专为云环境设计。我们的主要客户是那些在云环境中运行应用程序和基础设施的工程师。我们的服务适用于大大小小的公司。
So our smallest customers don't pass anything in their individuals or students. And our largest customers are the largest companies in the world, paying tens of millions a lot of a year. And you'll have pretty much everything in between. But very extremely technical product sold to a technical audience. And the core has been observability historically. What is happening in that space? It sort of feels like there was infrastructure monitoring, there were application monitoring, it sort of feels like things are starting to collide a little bit. Is that fair?
我们的最小客户通常不会涉及到个人或学生,而我们最大的客户则是世界上最大的一些公司,每年支付数千万的费用。在这两者之间,还有各种其他客户。我们提供的是一款非常技术化的产品,面向技术用户群体。历史上,这个产品的核心一直是可观测性。那么,这个领域现在发生了什么变化呢?看起来基础设施监控和应用程序监控之间似乎正在逐渐融合。这样理解是否准确?
Yeah, I mean, it used to be different categories, used to be called monitoring. A lot of the use cases are fairly similar. And it used to be Balkanized. So it used to be the used to be monitoring, application performance monitoring, network monitoring, log management, user experience monitoring. All of these were different categories with different user bases and different types of instrumentation, different vendors.
这段话的中文翻译可以是:
是啊,我的意思是,以前有不同的类别,叫做监控。很多使用场景都非常相似。而且以前是分裂的。以前有应用性能监控、网络监控、日志管理、用户体验监控等等。这些都属于不同的类别,针对不同的用户群体,使用不同的仪器和由不同的供应商提供。
I think what we've done that, to be honest, was kind of, I mean, it was actually the starting point of the company, but was a bit of a hard selling, surely, was bring together this category into one platform. The idea behind that adog was, well, those teams don't see it. I don't understand the world the same way.
我认为我们所做的事情,说实话,算是公司成立的起点,不过确实有点难推销,就是把这个类别整合到一个平台上。背后的想法是,这些团队看不到这一点。他们对世界的理解方式不同于我们。
I mean, for the story there, in the previous company, my co-founder and I used to run two different teams. I used to run the dev team and used to run the ops team. And even though we're very good friends, we have everyone on our teams in these companies because it was a fast growing startup. We did end up with ops that hated devs and devs who hated ops and fighting all the time and finger pointing.
在我的上一家公司里,我和我的联合创始人曾负责两个不同的团队。我管理开发团队,他管理运营团队。虽然我们是很好的朋友,但由于公司发展迅速,我们团队的每个人都参与了进来,结果导致运营和开发团队之间产生了矛盾。运营团队讨厌开发团队,开发团队也讨厌运营团队,双方经常争吵和相互指责。
So the starting point was, hey, let's get them in the same platform, showing you same language, the same view of the world and working together. So that, I mean, the first use case we brought up was really infrastructure monitoring for cloud. And then we added all of the other parts of that stack. So application, log management, user experience, network, all the other things that wouldn't make sense in there.
起初,我们的目标是将他们聚集在同一个平台上,使用相同的语言,共同展示对世界的相同视角并协同工作。第一个应用场景是云基础设施监控。之后,我们逐步加入了整个技术栈的其他部分,比如应用程序、日志管理、用户体验、网络等等,这些都是密不可分的组成部分。
And very much for anybody that has been following the data doctrine a little bit, what's been amazing to watch is that evolution of a time from that start to a platform that keeps getting broader and more horizontal as you keep adding product after product every year. And in fact, it seems so you have this chart in your public document somewhere where actually the number of things that you add every year features new products seems to be increasing, which is amazing to watch.
对于那些一直关注数据学说的人来说,令人惊讶的是,能够看到这种演变:从一开始到现在的平台,随着每年不断添加产品,平台变得越来越广泛和多样化。事实上,你们似乎在某个公开文档里有一张图表,其中显示每年添加的产品和新功能的数量似乎在增加,这种发展真是令人惊叹。
But going back to the beginning of that, so the vision was always to be a platform. Because that's that kind of cliche in the adventure and startup circles that you need to be a tool before your platform. So how do you navigate that? Well, I mean, initially, not very well. So the idea was, yes, we were going to be this platform that was always on there, that was in the way we started the company. And we had this vision of multiple data sets, multiple teams, multiple sets of use cases, all meeting into this platform.
回到一开始的想法,那时的愿景一直是要成为一个平台。在冒险和创业圈里,有一种老生常谈的说法是:你需要先成为一个工具,然后再成为一个平台。那么你该如何实现这一点呢?坦白说,一开始我们做得并不好。我们的想法是,要成为一个一直在线的平台,这是我们创建公司时的初衷。我们的愿景是,有多个数据集、多个团队和多种用例都能在这个平台上汇聚。
So we started the company, we were super excited, we applied to sorts of incubators, we got to a Y Combinator interview, and then we didn't get into Y Combinator. I have an email from a program that says, no platform is only as good as its first product, and you don't have a first product, la la la. So it was not like, you're right, like it actually was a tough sell. And to be fair, I mean, when we started raising our product, like the first beta of our product, we did call it a data platform, as opposed to calling it a very specific use case. And everybody loved the idea, like the users loved the idea. But people were not coming back to the platform, and also, but nobody was paying for it. So that was a bit difficult. At some point, we decided to name it monitoring, which was the name of the category before us, really, in fast-section monitoring. And without making too many changes to the product, we had a few small things to make sure it would be called that way.
于是我们创办了公司,特别兴奋,申请了各种孵化器,甚至获得了Y Combinator的面试机会,但最终没能进入Y Combinator。我收到一个项目的邮件,上面写着:“没有一个平台能好过其首款产品,而你们还没有首款产品,等等。”这确实是利用难度很大的事情。说实话,当我们开始推出产品的时候,我们确实把它称作一个数据平台,而不是一个非常具体的应用场景。所有人,包括用户都很喜欢这个想法,但他们没有重复使用这个平台,而且也没有人愿意为此付费,所以这有点难。后来,我们决定把它命名为监控,这其实是我们之前所在领域的一种分类名字。我们对产品没有做太多变化,只是做了一些小调整,以确保可以称它为监控平台。
Immediately, it got traction. Immediately, people understood, okay, this is why I need to bring it back, and this is how I convinced my boss I need to pay for it. And we were off on the races from now on. Because there was a known budget item. Yes, and that comes back, we can talk about it later, but in terms of understanding where you're going as a platform, but also who you bring that back to where your customer bases today, I think it's a key part. You actually have to take your customers where they are, and talk to them in terms of the understanding, in terms of their existing categories, their existing span, their existing solutions.
立即,这件事引起了关注。人们立刻明白了,这就是我需要重新引入它的原因,这也是我说服老板为它买单的理由。从那时起,我们就开始顺利运作了。因为这是一个已知的预算项目。是的,这个我们可以稍后再谈,但就理解平台的未来发展方向以及如何将其重新引入到当前的客户群而言,我认为这是一个关键部分。你必须根据客户当前的情况,与他们沟通,用他们已经熟悉的类别、预算和解决方案进行交流。
So maybe walk us through some examples of that evolution over the years. You started with metrics, trace, logs. What was the next few products over the years? We started with just metrics and infrastructure. We didn't have tracing, we didn't have logs. And for the first seven years of the company, we didn't do anything else. We had the great chance of having amazing interaction with that core product. So we were just too busy keeping up with the customer base, both in terms of scaling the systems, but also just adding all the functionality they needed for that, and supporting all these new customers. So for the first.
可以跟我们讲一下这些年产品演变的例子吗?你们一开始做的是指标、追踪和日志。接下来这些年又推出了哪些产品呢?我们最初只做指标和基础设施,没有追踪功能,也没有日志。在公司成立的前七年,我们只是专注于这些领域。我们有幸能够与核心产品进行深入的互动,因为我们太忙于应对客户需求了,不仅是在系统扩展方面,还要添加他们所需的各种功能,并支持所有新客户。所以在最初的几年里,我们的重点一直在这里。
Metrics is just any number for performance of your infrastructure. Yeah, so metrics, we did that. We had a number of integrations, basically that would get the data from whatever systems our customer were using, whether that's their cloud provider, the database they're using, the operating system, the CDN. But also we would let them, we still do, submit custom metrics for their own applications. So if they want to track, for example, the number of times a certain function is called, then write terms in shop, write them in shopping cars, do a lot of amounts of adding pressures, they can do all that. And it did a lot of it. That's what made the product so successful, like that we could cover the gamut from the, would the, how much CPU you have, all the way to how much revenue you're making with the product. That was extremely appealing, especially to all of the digital native companies at the time that were moving into the cloud. So we started with that, I would say until from 2010 to 2016, we mostly did that. But our platform was still fairly open. You could add any sorts of data, manipulate the data in all sorts of interesting ways, build analysis and visualizations and things like that. And what we saw was that a number of our customers started building other products for themselves on top of our platform.
指标只是用于衡量您基础设施性能的任何数字。是的,我们确实做了有关指标的工作。我们与多个系统进行了集成,以获取客户正在使用的各种系统的数据,无论是他们的云服务提供商、使用的数据库、操作系统还是内容分发网络(CDN)。同时,我们还允许客户提交他们自己的应用程序的自定义指标。比如,他们想跟踪某个函数被调用的次数、购物车中的条款书写量或大量的添加压力等操作,他们都可以做到。正是这种功能,使我们的产品非常成功,因为我们能够从 CPU 的使用量到产品带来的收入等各个方面提供数据支持。这对那些正转向云服务的数字原生公司极具吸引力。从 2010 年到 2016 年,我们主要专注于这一领域。我们的平台依然很开放,用户可以在其中添加各种数据,以各种有趣的方式处理数据,进行分析和可视化等。此外,我们还观察到,一些客户在我们的平台上为自己构建了其他产品。
So we didn't do application performance monitoring, there were other products that were doing that, like a whole category of products. But we saw that a number of our customers were building a poor managed APM, like that's the shorthand for application performance monitoring into our platform. And that told us a few things that told us, well, does it need? And, but also our customers believe that we're part of the solution. So they want to see it there and they're willing to go if we do trouble of hacking together something on top of us. So that gave us great confidence that our initial vision was good and that we should keep developing that. And the next product should be application performance monitoring and we started building that. And when was that what year? 2016 I think is when we engaged with that. And this one actually took us a while to get right.
我们最初没有进行应用性能监控,因为当时已经有其他产品专门负责这类功能,那是一个完整的产品类别。然而,我们发现一些客户在我们的平台中整合了一个管理不善的应用性能监控(简称APM)。这揭示了几个问题:首先,是否真的需要这样的功能,而我们的客户似乎认为我们能成为解决方案的一部分。他们希望在平台中看到这样的功能,并且即便需要自己动手整合也愿意这样做。这让我们对最初的愿景充满信心,并促使我们继续发展。于是我们决定下一个产品就该是应用性能监控,并开始着手研发这个功能。具体是在哪一年开始的呢?应该是2016年,那时我们着手进行这项工作,但需要花一些时间才能做到完善。
And we can also come back to that, but this was a part of it was going from product number one to product number two, which is hard. Part of it was also that it was, it's actually a category that has very high table stakes and it is a bit of a slow grind to get everything going with customers. So we took us several years actually to get that product to be truly successful. Yeah, and then there was a new relic of dynamics. Yeah, there were a number of other companies out there. And at about the same time, we also found that, blog management also is a category that belongs into what we're doing. It's another aspect of observability that is very important for users. And then, same thing, we saw customers trying to hook their log management to us in different ways and build hack solutions for doing that. We also found that the problems the customers have didn't stop at the barrier between application and infrastructure and a lot of the back. They actually cross everything. So it made complete sense to everything one goal. In this one, log management, so we started working on that too. This one we accelerated with M&A. So we actually bought a company in France. It's a small company that has an early product for that. And we accelerated the development. I think through the combination of this being accelerated by M&A, this being also an easier product to get to a critical mass compared to APM. We actually ended up with both the interaction at exactly the same time, even though we started with one, maybe two years after the other. What came after? There was synthetics. Yeah, we did. The rest of what's part of an observatory stack. So we did synthetic testing, which is automated testing for APIs or application, things like that. We did really awesome monitoring, which is measuring what end users are actually doing in the application, first for performance reasons, but then increasingly for business analytics reasons. So what are my users clicking on? What are they going from there? That kind of stuff.
我们可以回到这一点,但这个话题的一部分是从产品一转到产品二,这非常困难。还有一部分原因是这个类别本身的竞争非常激烈,需要慢慢地与客户建立关系。所以我们花了好几年才让这个产品真正成功。同时,还有一些新的动态出现,比如有其他公司竞争,我们也发现日志管理领域正好符合我们的业务范畴,这对于用户来说在可观测性方面也非常重要。后来我们发现客户尝试不同方式将日志管理与我们的产品联系起来,并进行一些实用的解决方案。我们认识到,客户的问题不仅局限于应用程序和基础设施之间,而是横跨了一切。所以,我们的目标是整合所有这些内容,为此我们也开始着手于日志管理。我们通过收购加速了这一进程。我们在法国收购了一家小公司,它有一个早期的产品,我们加速了它的开发。我认为由于收购的推动,加上比应用性能管理(APM)更容易达到临界质量的特点,我们实际上在同一时间推出了两项产品,尽管我们可能是在另一项之后一两年才开始的产品。接下来,我们涉及了合成(synthetics)。是的,我们进行了观察栈(observability stack)的其余部分。我们开发了合成测试,这是针对API或应用程序的自动化测试。此外,我们还进行了出色的监测,测量终端用户在应用程序中实际的操作,一开始是出于性能的原因,后来越来越多是为了业务分析,比如用户在点击什么,他们从那里去哪等等。
We started expanding into security. So entering, doing security on top of logs, you know, there's a category that's called SIEM for that. We started doing security on cloud environments, so both on the infrastructure side and the application side. And we have a thesis that security, like five years from now, will be, or rather let me rephrase it, it will be a no brainer that you have to attach your security to your observability, because that is what is, get deployed everywhere in your application, in your infrastructure, and it would get used by all your engineers. And to solve your security issues, you cannot just rely on a team of, say, 10 security engineers, you need to rely on your 50 ops engineers and your 200 software engineers to make that happen. That's because a threat would manifest into logs, into activity, or. Yes, but also, like most of the security issues are introduced by your development team and your operations teams, and most of the fixes have to be made by your development team and your operations teams. Like mostly dealing with security is not, you know, monitoring North Korean hackers. I mean, there's a bit of that, but mostly it's about patching all of the various vulnerabilities you have, because maybe you had a mistake when you were coding, or maybe you use a library and that library now has a vulnerability and you need to upgrade your code and make sure everything works. Or you misconfigured something, or there's a vulnerability that exploits certain type of configuration. So you need to go and continuously go and close all of those vulnerabilities, and the people who do that are typically not the security team, they're the development and the operations teams.
我们开始涉足安全领域。在日志基础上进行安全工作是属于SIEM(安全信息和事件管理)这个类别的。我们也开始对云环境进行安全防护,包括基础设施和应用程序两方面。我们认为五年后,将安全与可观测性结合在一起将成为理所当然的趋势,因为这将应用于你所有的应用程序和基础设施中,并被所有工程师使用。要解决安全问题,不能仅依赖一个由10个安全工程师组成的团队,而需要依靠50个运维工程师和200个软件工程师的力量。这是因为威胁往往会体现为日志或活动记录。而且,大多数安全问题是由开发和运维团队引入的,而大多数修复也必须由他们完成。安全管理并不主要是为了监控来自外国黑客的攻击,尽管这也是一部分原因,主要工作是修补各种漏洞。因为可能在你编码时出现了错误,或者你使用的某个库现在有了漏洞,你需要升级你的代码并确保一切正常,或者是配置错误,或某种配置被恶意利用。因此,你需要持续地去修复所有这些漏洞,而执行这些工作的通常不是安全团队,而是开发和运维团队。
So it's very interesting, right? The first two or three, so going into APM and other things, sounds like your customers basically dragged you into it, they started building the product that you didn't have and showed you the way. Is that true as well as security? Was that more of a strategic kind of decision? Because I totally get how a lot of this manifests in what you monitor, but equally it's a different industry, it's a different buyer. It's a big oleap, I think it's true in part, let me simply with customers build some versions of that themselves. And for some parts of what we do, it's a natural evolution, like in log management, for example, the companies that used to sell logs before us, then evolve into security companies as part of that. So it's understood that the two sort of leave together.
所以这很有趣,对吧?前两三个产品,像是进入APM(应用性能管理)和其他领域,听起来是因为你们的客户基本上把你们拉进去了。他们开始构建你们当时还没有的产品,并给你们指引了方向。这和安全领域也是一样的吗?这是一个更具战略性的决定吗?我完全理解这些如何体现在你们的监控内容中,但同样地,这是一个不同的行业,面对不同的买家。这是大的跨步,我认为这部分是对的,让我简单说,就是客户自己构建了一些版本。而对于我们所做的一些部分,比如日志管理,这是一种自然演化。例如,那些曾经销售日志的公司,后来演变成安全公司,因此,这两者通常是一起发展的。
But for other parts, application security and cloud security, for example, these were very separate. So I think it's a bigger shift, so it's also a shift in terms of who to think about the security market in general. So I mentioned earlier that observability used to be very fragmented and now is one big category, really, that's what we're leading today. Security is still an extremely fragmented category. I mean, you're in VC, so you probably can spend the first three hours every month there, keeping up with all the new funded companies and cyber security. Yeah. And you can have an entire investing career just doing security, which some people do. Yes, there's so many of them.
但在其他方面,比如应用安全和云安全,它们以前是非常独立的。所以我认为这是一个更大的转变,也是对整个安全市场的思考方式的转变。就像我前面提到的,可观察性过去是非常分散的,但现在已经成为了一个大的类别,这就是我们今天所引领的。然而,安全领域仍然是一个非常分散的类别。你在风险投资领域,所以你可能每个月头三个小时都在了解所有新成立的公司和网络安全方面的动态。实际上,你可以把整个投资生涯都专注于安全领域,有些人确实是这样做的。是的,相关公司实在是太多了。
And as a result, you end up having so many different sub-categories. And we think that it's too complex. Like it's impossible for the customers to actually understand how to piece that together and to integrate everything into a consistent hole that really protects them. And so we think that security is going to go through the same consolidation, basically, into platforms with some extra products here and there, but mostly relying on large platforms. I'm very fascinated by that topic of how you keep building products, because as you said, it's so hard to get a second product. Most companies never get the second product.
因此,最终你会有很多不同的子类别。我们认为这太复杂了,客户几乎不可能理解如何将其拼凑在一起,并整合成一个真正保护他们的一致整体。所以我们认为,安全领域也将经历同样的整合,基本上会转向以大型平台为主,辅以一些额外产品。我对如何持续开发产品这一主题感到很着迷,因为正如你所说的,获得第二个产品是非常困难的,大多数公司从来没有推出过第二个产品。
So one aspect is your customers point away, so that's super interesting. Sort of almost logistically, how long before you jump into an industry and do you start planning that? Like, how does that start? You do research efforts. You have a consulting team, or is that USU decides this? And then how long do you plan before you start building and then launching? Well, mostly we hear about it from customers. So we have this strong bias in the company that are windowing to the world is our customers. So for example, when we think about competitions, or competition in general, we don't spend a lot of time reading our competitors' possibilities or website. Instead, we talk to our customers and we hear what they have to say about it, whether it's registered with them or not, which seems to be valuable to them or not.
所以,有一个方面是你的客户的需求,这非常有趣。从某种程度上说,在进入一个行业之前,你会在多长时间开始计划?这一过程是如何开始的呢?你会进行研究工作吗?你有一个咨询团队,还是由公司自行决定?你会计划多长时间才开始构建和推出产品?通常,我们是从客户那里得知相关信息的。我们公司有一个强烈的倾向,即客户是我们了解世界的窗口。所以,例如,当我们考虑竞争或竞争者时,我们不会花很多时间去研究竞争对手的动向或阅读他们的网站。相反,我们与客户沟通,倾听他们对这些事物的看法,以及这些事物是否对他们有吸引力或价值。
Basically, the world around us exists for the prison or our customers. So from that, we get a sense of what's actually a problem for them, what seems to be real. And then we decide what we might try to go and walk on. But the way we build it is so we don't do it like Apple. We don't disappear in a basement for three years, and then ship a fully-formed product that takes the world by storm. Instead, from the earliest days, we work with design partners, we work with customers, and we try to ship something to them as quickly as possible. So that's really the way we develop. And then we trade.
基本上,我们周围的世界是为了我们的客户而存在的。因此,我们可以了解到他们真正的问题是什么,什么对他们来说是现实的。然后我们决定尝试解决哪些问题。但是,我们的开发方式与苹果不同。我们不会在地下室消失三年,然后推出一个震撼世界的成熟产品。相反,我们从最初阶段就与设计合作伙伴和客户合作,尽快给他们提供一些产品。这就是我们的开发方式,然后我们再进行优化和改进。
And then we trade. And you offered for free initially for those design partners? Well, there's a whole process. And actually, a key part of building a new product is understanding what's valuable and what's not. And it's difficult because when you start and your design partners are typically your existing customers because you have strong relationship with them. And typically, they love you. They love your product. So they're happy to spend time on working on new things. But they haven't necessarily thought through the whole value of what it is they're asking you to build. So the way we do it is we start by building with them. So we ask for what the problems are. We get a sense of what else they might use for it. Was their next best alternative or what they were using before? That's not good enough. So it helps ground everything. But then as soon as we have enough product, we basically say, OK, it's going to cost you this much to use this product.
然后我们进行交易。你最初是免费提供给这些设计合作伙伴的吗?其实,还有一个完整的流程。事实上,开发新产品的关键部分是弄清楚什么是有价值的,什么不是。这并不容易,因为当你开始的时候,你的设计合作伙伴通常是现有的客户,因为你们之间有牢固的关系。通常,他们非常喜欢你和你的产品,所以他们很乐意花时间参与新产品的开发。但他们并不一定完全考虑过他们要求你构建的东西的全部价值。因此,我们的做法是先与他们一起开发产品。我们会询问他们的问题是什么,并了解他们可能会怎样使用这个产品。他们下一个最好的选择是什么,或者他们之前用的是什么,而这些选择都不够好。这有助于我们打好基础。但一旦我们的产品足够成熟,我们基本上会告诉他们,使用这个产品需要多少费用。
And what typically happens at this stage is that when the product is ready, when it's great, about half of the design partners just disappear. The people who are showing up in the meeting every week or twice a week and were very happy to work with us, they start ghosting the product teams because they realize, actually, I'm not going. I'm not able to pay for it. I don't want to pay for this. It's not valuable enough. And so that's the first gate. The first gate is do you retain enough of the design partners? Is there enough value in what we're doing there? Or is it just good stuff?
在这个阶段通常发生的情况是,当产品准备好了、变得出色的时候,大约一半的设计合作伙伴就消失了。那些每周出席一次或两次会议并且很乐意与我们合作的人,开始对产品团队置之不理,因为他们意识到,实际上他们不打算购买,也无法支付这笔费用。他们觉得产品不够有价值。因此,这是第一个关卡:你是否能保留足够多的设计合作伙伴?我们所做的事情有没有足够的价值,还是仅仅是一些不错的东西?
After that, we start opening up the products more broadly. So we typically are going to have some form of a private or public beta for the product. And then we're going to go through another and initially for free. And then we're going to go through another phase of, OK, so now we're going to announce pricing. And we're going to start charging the most active of those customers. And same thing we see, do we have enough retention there? Do we clear the bar of that product being valuable enough that we can keep going? And then we do that one more time, which is when we completely open it.
在那之后,我们开始更广泛地推出产品。通常,我们会为产品进行某种形式的私测或公测。最初,我们会让大家免费使用。接下来,我们进入另一个阶段,宣布定价,并开始向最活跃的用户收费。同时,我们会观察用户是否足够留存,看看产品的价值是否足以支持我们继续前进。然后,我们再进行一次这样的测试,直到我们完全开放产品。
And when we start charging automatically for usage, we see, OK, so do actually, when people start using it, do they keep using it after when we start charging for it or not? And then that tells you, OK, the value is there or the value is not there. And I think it's very important. We feel that the worst that can happen in software is that you build stuff, but nobody cares about it. And it's very easy to build stuff. Have you killed products that did not meet those bars?
当我们开始自动对使用进行收费时,我们会观察:当人们开始使用它后,当我们开始收费时,他们是否继续使用?这个过程可以帮助我们判断这个产品是否有价值。我认为这非常重要。我们觉得软件领域最糟糕的情况就是你开发了产品,但没有人关心它。而开发产品其实很容易。那么,你是否会停止运营那些未达到预期的产品?
We did not kill products. But the way we do it is we start small. And we've waited a long time to scale up teams or products. As they were still searching for basically the core value or we would be able to sell in the end. So some of these are still searching for the exit. Some of these are still. We have a small effort going forward exploring. And many of those have scaled as we went through all those gates. And we validate the value. And we understood better the packaging.
我们没有淘汰产品。我们的做法是从小规模开始。我们通常会等待很长时间才扩大团队或产品规模,因为在此期间,我们还在寻找产品的核心价值,或者确定最终能否将其销售出去。所以,有些产品仍在寻找出路,有些则仍在持续探索中。我们在前进过程中经过了一系列考验,很多产品因此实现了规模化增长。在此过程中,我们验证了产品的价值,也更好地理解了产品的包装方式。
And we could then have a very clear roadmap in terms of what we need to build for those customers to keep scaling. Who does this? When you launch a new product, do you have an internal, I don't know, sure, part team? I know you're active on the M&A front. So like I assume in some cases it's whoever you buy. But do you take people from other products to reassign them? How does it work? Yes. And that's part why it's difficult to build multiple products.
我们可以制定一个非常明确的发展路线图,明确我们需要为这些客户建立什么来保持扩张。那这是谁负责的呢?当你推出新产品时,你是否有一个内部的团队来处理这些?我知道你在并购方面很活跃,所以我猜想在某些情况下是由你收购的公司来处理。但你会从其他产品中抽调人手重新分配吗?这是怎么运作的呢?是的,这也是为什么开发多个产品是一件困难的事情的一部分原因。
Because what happens when you start thinking about product number two typically is you have product number one that is very successful. But if it is very successful, chances are everybody is super busy just keeping up. And everybody that is super good and you would want to trust with starting a new product is load bearing on your core product. So that's difficult. You need to pull people away. And that's painful. That's hard. The second part is that scaling a successful product and starting a new product are very different motions.
当你开始考虑开发第二个产品时,通常的情况是你已有的第一个产品非常成功。但如果它真的很成功,那么大家可能都特别忙于维持它的运作。而那些你信任,并愿意让他们负责开发新产品的人,往往也是你核心产品的关键人才。因此,这就很困难。你需要从现有团队中抽调人员,这个过程非常痛苦且艰难。其次,扩展一个成功的产品与启动一个新产品是完全不同的工作方式。
And I would say people feel very differently about it. Like in one situation, you mostly walk into situations where customers love you and you know exactly what you need to do next. And you can work with them. In the other situation, like you don't have traction yet, you're trying to understand why. And you have to understand, you have to read the decipher, the cryptic feedback you're getting from customers. Because again, these customers in general, everybody are people who are good people. They don't want to hurt your feelings. So when you talk to them, they'll say, oh, yeah, yeah. No, this product is great. It doesn't actually work. But it's great.
我想说,人们对此的感受差异很大。在某种情况下,你大多会遇到喜爱你的顾客,你清楚下一步要做什么,并且可以与他们合作。而在另一种情况下,当你还没有获得足够的吸引力时,你需要弄清为什么会这样,你必须解读顾客给出的隐晦反馈。因为总的来说,这些顾客都是好心的人,他们不想伤害你的感情。所以当你与他们交谈时,他们可能会说,“哦,是的,是的,这个产品很棒”,其实心里想的是“这产品根本不行,但还是很棒”。
And the people who are used to scaling the products that work ignore the negative part, whereas it's the only part. That's worth listening to. And I assume that's true on the sales side as well. You can become super great at selling something that people want. And then you have to sell the new thing. So how does that work? That part I would say for us is a bit easier, because we get adopted bottom up. So we focus on getting usage first, so making the product discoverable, getting really short time to value inside the platform, in the product itself.
习惯于扩展成功产品的人往往会忽略那些负面的方面,但实际上,那才是值得关注的部分。我猜在销售方面也是这样的。你可以非常擅长于销售人们需要的产品,但若要销售新的产品,又该如何适应呢?对我们来说,这一部分相对简单一些,因为我们的产品是从下往上被用户接受的。我们专注于先提高使用量,让产品便于发现,并在平台和产品内部实现快速的价值呈现。
So then the sales side of things is a bit easier. I think as the products get more mature, and as the large enterprises start using them more, it does more of a sales job, basically, to work on consolidation. So basically, situations where customers were using 12 things before, and they're just going to use us after. So there's more of a sales job to do to be done there. That's more traditional. But otherwise, we mostly do it bottom up.
因此,销售方面就变得相对容易一些。我认为,随着产品的逐渐成熟和大型企业的更多使用,它实际上会起到更好的销售作用,主要是在巩固方面发挥作用。简而言之,以前客户可能使用了12种不同的产品,现在他们会选择只用我们的产品。所以在这方面有更多的销售工作要做,这是一种更传统的方式。不过,我们大多数情况下主要还是从下到上开展工作。
And one last thing to mention, by the way, on putting people into new projects is that people need to see through the angle of people caring for their career, too. You have to make sure it's safe to work on the product. It's not successful yet. Because otherwise, people just want to stay on the start of the show and not go into that thing, and they don't know if it's going to take off or not. Now, how do you measure the success of a new product? Do you have an internal metric? I don't know, around like a tax rate or whatever you call it? I mean, we tried to get very good signals. So I mentioned that we established value by starting to charge for products initially. So we try and not do too much bundling.
顺便提一下,把人们安排到新项目上的最后一点需要注意的是,人们也需要从关心自己职业发展的角度来看待这个问题。你必须确保在产品上工作的安全性。因为这个产品还没有成功。否则,人们只想留在显眼的位置,而不愿意进入这个新项目,他们不知道是否会成功。那么,如何衡量新产品的成功呢?你们有内部的指标吗?比如说,像是税率之类的?我们努力取得良好的信号。我提到过,我们通过一开始就对产品收费来建立价值。所以我们尽量不进行太多的打包销售。
Of course, you do some of it, because it's an enterprise software. You can't just piece out everything, every single feature. But we try to get clear signals in terms of is it getting adopted, is it getting used more and more? So both on the revenue side, but also on the straight activity into the product. So how many users do they have? What's the footprint in terms of the data sets that are being sent? The impact on infrastructure, all those things. So we track all of those. We also optimize for very short feedback loops. So in particular, and that dates back from the early days of the company, we always start with very low commitments and very short timeframes.
当然,作为一款企业软件,有些功能是需要我们自己去完成的,不可能把每一个特性都模块化。不过,我们会努力获取明确的信号来了解它是否被采用,以及使用率是否在不断增加。因此,我们不仅关注收入方面,还关注产品的实际使用情况。例如,有多少用户在使用?数据集的覆盖范围有多大?对基础设施有什么影响?我们都会对这些因素进行追踪。同时,我们也优化了非常短的反馈循环。这一做法源自公司早期阶段,我们总是从非常低的承诺和非常短的时间框架开始。
So for new products and new companies, I would argue month to month is great. Because your customers can't try at any time, which means you'll get the hard reality to hit you in the face. And you can't ignore it, which is a problem when you have. So you say you sell one year, three year deals. You can, even though you might see concerning adoption or usage metrics, you can fool yourself into thinking that you'll be able to fix it. So when you have a very short cycle time on that, you really have to fix things very quickly.
对于新产品和新公司来说,我认为按月进行评估是非常好的。因为你的客户可以在任何时间进行尝试,这意味着你会面对现实,并且无法忽视这个问题。相反,如果你销售一年的或三年的合约,即使你看到产品使用或接受度有问题,你也可能会误以为能有时间去解决。但如果是很短的周期时间,你就必须非常快速地解决问题。
And is that just an impression that you keep releasing one more product? Is that like on the slides that I was mentioning earlier, or is that you've just built a muscle and you're just cranking because you know how to do it and you keep doing it at the industrial scale? Well, it's more effective of demand. So I think we see that there's a lot more we need to do in observability. And there are other categories I mentioned. Security, there's a number of new things about AI, of course. We'll have to talk about it at some point. Yes. Right after this coming up. There are things that are getting closer to developer too, that are very interesting.
这只是你们不断发布新产品的印象吗?还是说就像我之前提到的幻灯片中那样,你们已经练出了这个本事,能够持续不断地大规模生产?其实,这更多是因为市场需求。我们发现,在可观察性方面还有很多事情需要做。此外,我提到的安全性、以及关于人工智能的一些新内容,当然也需要在某个时刻进行讨论。是的,马上就要谈到这些了。还有一些更接近开发者的东西也非常有趣。
Yeah, it's a thought that came to mind as you were describing. The fact that security is a developer problem. It sort of feels like that's a world of like this, the set ups. You've been very focused on the on the metrics and what comes out of the machines, but it sort of feels like you need to go into the world of code. Is that maybe already doing that, but is that part of the idea? I mean, part of the idea is you have to tie it back to what the developers are doing. Yes. I think the act of coding itself historically hasn't been a narrative that's conducive to being solved with software products so much. I think it tends to be more the community side of the world as opposed to everything that relates to production systems. But we think it's a we definitely need to close the loop between what's happening with developers are doing on the type of line of code and what the impact is actually going to be on the application in production, on their end users, on their business, on the security posture of their business, like this is the hard part.
是的,这个想法是在你描述时浮现在我脑海中的。安全问题其实是开发者的问题。这种感觉就像置身于这样的一个世界,你一直专注于指标和机器的输出,但似乎需要深入代码世界。可能你已经在这么做,但这也是其中的一个想法吗?我的意思是,部分想法是你必须将其与开发者的工作联系起来。是的,我认为编码本身在历史上并不是一个容易通过软件产品解决的问题。我认为这更多地与社区相关,而不是与生产系统有关的一切。但是我们确实认为有必要在开发者所写的代码类型和代码在生产环境中应用所产生的影响之间形成一个闭环,影响终端用户、业务,以及他们业务的安全状态。这就是难点所在。
And you know, again, we think we're meeting, I'm getting a little bit ahead of myself there in terms of the, the, where AI is going to take us. But one way we like to think about it is the, the wave of the, the exponential increase of developer productivity. If you go back 40 years, 50 years, people were coding in a machine language on a credit card, like my dad started coding on. It was like rulers as well, right? Yes, rulers if I had, yeah. So fairly low productivity. Then you know, you went, you could on on keyboard and screen, but still on a machine. Then you had more advanced languages, then you have more advanced languages and libraries that other people wrote that you could use, but you still needed to pretty much write all the code yourself and, you know, buy books from the library to understand what to do.
你知道的,我们认为我们正在迎接一个新的时代,我这里稍微超前了一点,就是人工智能将带我们走向何方。但我们喜欢用一种方式来思考这个问题,那就是开发者生产力的指数级提高。回顾40年、50年前,人们是在像我的父亲那样用信用卡大小的机器语言进行编码。那时还像是用尺子来编码,对吧?是的,如果有尺子的话,就是这样。所以生产力相对较低。然后,你知道的,你可以在键盘和屏幕上进行操作,但仍然是在机器上。后来有了更高级的编程语言,然后又有了更高级的语言和其他人编写的库,你可以使用这些库,但你仍然需要自己编写所有代码,并且需要从图书馆买书来了解该怎么做。
Then you had the internet, then you had open source with all of the libraries you could use everywhere. Then you had SAS and pass and basically all of those things you can just plug into the API's that are going to do a lot of the work for you. So we've seen over the past 40, 50 years, orders of magnitude of productivity increases. And I think AI on top of that is going to give us maybe another order of magnitude or two in terms of what a human can produce functionally.
首先有了互联网,然后出现了开源技术,提供了各种可以随处使用的库。接着有了SAS和PASS,基本上这些东西你只需接入API,就能完成大量工作。因此,在过去40到50年里,我们目睹了生产力的大幅提升。而我认为,人工智能能在此基础上,再为人类的实际产出增加几十倍。
The flip side of that though is every time you add more complexity, or every time you add productivity, you add complexity, meaning that you have less and less of an understanding of what it is you're doing. Because I mean, you just, you did something super complicated in five seconds. You can't possibly know what's going to happen on the back of that. So a lot of the value shifts from just creating that thing to understanding how it's going to behave, how it's changing over time, what its failure modes are, what impact it has on its users, and basically how it can be abused from a security perspective. And that's where we see ourselves playing a role in the long term.
翻译成中文,并尽量易读:
但另一方面,每当你增加更多的复杂性、或者提升生产力时,其实你也在增加复杂性,这意味着你对自己正在做的事情理解得越来越少。因为你可能在五秒钟内完成了非常复杂的事情,你不可能完全知道接下来会发生什么。所以,很多价值开始从仅仅创建那个东西,转移到理解它将如何表现、如何随着时间变化、故障模式是什么、对用户有什么影响,以及从安全角度来看如何防滥用。这就是我们长期以来看到自己的定位。
So as I think about it, there's different themes for data targeting AI. You touch on a couple of those. There's AI friend of foe for data targeting business. There's building AI into data dog products to make them better, faster, smarter. And then there's probably a lesser but interesting theme around how data dog employees use AI to maximize performance productivity, all those things. So maybe starting with the first one.
当我思考这个问题时,我认为数据瞄准AI有不同的主题。你提到了一些。其中一个是AI在数据瞄准业务中是朋友还是敌人。还有一个主题是将AI构建到DataDog的产品中,使它们更好、更快、更智能。最后,还有一个可能较少但有趣的主题,即DataDog员工如何使用AI来最大化他们的表现和生产力。那么,也许我们可以先从第一个主题开始。
So I, it maybe playing it back does feel like the increase in complexity is your friend. So the fact that we may have all those machines that will do things for us that we may or may not understand is a good thing. Do you think there is a world just to play a little bit like a G.I. Duma where AI actually becomes a problem for data dog in, I don't know, automating or being able to do all the things in a way that where humans do not need to be involved? Oh, but I think you at some point someone is to control the AI in some form, right? So, or maybe not, maybe we just, you know, we'll be.
所以,我觉得回想一下,复杂性的增加其实对我们有利。那些自动为我们工作的机器,无论我们是否理解它们,这都是一件好事。你认为会不会有这样一种情况,就像科幻电影中的情节,人工智能实际上成为数据领域的问题,比如在自动化方面能够做到让人类不需要介入?哦,不过我认为在某种程度上,人类还是需要控制人工智能,对吧?或者也许不需要,也许我们就会顺其自然。
Yeah, we'll all that's in the end, you know, but I don't subscribe to that to that region of the future. But I do think that the idea of the day, I do see that as one more evolution in the history of innovation, which is we just do more. And because we can do more more easily, we do even more. And then we need to manage it and understand it. I think that's the overall arc of things. And that's where we have potentially a never big on role, a never big on role to play in the future as it happens.
是的,我们最终都会走到那一步,但我不认同那种对未来的看法。不过,我确实认为今天的这个想法,只是创新历史中的又一次演变。我们能做的事情更多了,因为我们更容易做到,于是我们会做得更多。然后,我们需要管理和理解这些事情。我认为这就是事情发展的总体趋势。在未来的发展中,我们有可能扮演一个重要的角色,一个前所未有的重要角色。
And you know, when you think of the impact on our business, you know, there's a few ways to think about it. The most straightforward is, and the one happening right now is it just the emergence of AI just pushes more digitization and move to the cloud, you know, because, I mean, to capitalize on AI and your data. So it needs to be digital. And you probably are not going to build your data center for AI yourself. If you are a handful, maybe 10, 20 companies, yes, you are, because you are at such large scale, you're in the business of providing your services for others. But otherwise, you're not because you don't know what you need.
翻译成中文:
当你考虑到对我们业务的影响时,有几种方式可以想到。最直接的方式,也是现在正在发生的,就是人工智能的出现推动了更多的数字化和向云端的迁移。因为要充分利用人工智能和你的数据,它需要是数字化的。而且你可能不会自己建立用于人工智能的数据中心。除非你是少数,比如说十到二十家公司,因为你们规模如此之大,是在为其他公司提供服务。然而,其他的公司就不会这样,因为你不知道自己需要什么。
You, I mean, whole wheat companies even know today what to buy and what it looks like three years from now when they when they've realized those investments. That's impossible. This seems to be a little bit of trend around actually let's not move data to the computer to data to the AI, but bring AI to the data and therefore actually on prem or VPC to some extent is a good place to do AI, you know, and sometimes open source, hence, you know, a part of the NVIDIA rise, but also Dell and, you know, to be clear, the, what we call on prem at this level is the ground.
你知道吗,我的意思是,即使是全麦公司今天也不知道三年后该买什么以及这些投资会产生什么效果。这基本上是不可能的。现在似乎有一个趋势,就是不要把数据移动到计算机或AI那里,而是把AI带到数据上。因此,在某种程度上,实际上在本地部署(on-premise)或虚拟私有云(VPC)环境中运行AI是一个不错的选择。而且,有时候开源软件也很重要,这也是NVIDIA的崛起以及戴尔相关业务的一部分。但要明确,我们在这个层面上所说的本地部署其实就是地面。
Yeah. Like it's on prem, but it behaves, it looks like and it behaves exactly like the public cloud switch is the same thing. And there's a dynamic also right now where the compute itself, the GPU's are way, way, way, way more expensive and don't need that much bandwidth compared to everything else. So it's actually okay if, you know, your data is in Australia and your GPU is in the US. That's not a big issue.
好的。就像是在本地部署,但它看起来和运行起来完全像是公有云一样。现在还有一个动态变化,就是计算本身,尤其是GPU变得非常非常非常昂贵,但相比其他一切,它不需要那么多带宽。所以实际上,如果你的数据在澳大利亚而GPU在美国,这也没什么大问题。
That's not necessarily how things are going to be in the long run. So I think it's more of a side effect of where we are right now, you know, in terms of the technical solutions, the evolution, this has the models and the press of the GPU and all those things. So I don't decide anything. This is long term trend. And from your perspective, all of this are just systems and machines that speed out machine data that needs to be monitored, right? So for example, I was, you know, reading somewhere that you integrate with whatever NVIDIA part of the NVIDIA platform that enables you to monitor the health of GPUs.
这并不一定是长期的发展趋势。我认为这更多是由于我们当前所处的技术解决方案、技术演变、模型发展以及GPU等方面的暂时性结果。所以我不认为这代表了一种长期趋势。对于你来说,这一切只是需要监测的系统和机器数据。例如,我曾在某处读到,你们可以与NVIDIA平台的一部分进行集成,从而监控GPU的健康状况。
Yeah. Yeah, I mean, look, again, it's straightforward. That's more infrastructure. So we need to understand the GPUs. We need to understand there's new components in there, like there's models themselves from the outside of their components with latency and error rates and costs and things like that. We need to monitor the new databases and you vector databases and things like new. The whole new stack basically, around AI. But a vector database does not behave any differently than all app database from your perspective. No. And also the AI applications are typically the part that's a model in GPUs and you're a small part of that app, like the rest is, you know, it talks to a database and it talks to a web server and it talks to its source files and all that stuff.
是的,我的意思是,再次强调,这很简单。这就是更多的基础架构。因此,我们需要了解GPU,并且知道里面有新的组件,比如从外部看模型本身是带有延迟、错误率和成本等组件。我们需要监控新的数据库和向量数据库以及一些新的东西。整个围绕AI的新技术栈。但是,从你的角度来看,向量数据库的行为与传统的OLAP数据库并没有什么不同。而且,通常来说,AI应用程序中主要部分是GPU上的模型,而你在这个应用程序中只是很小的一部分,其余的部分需要与数据库、网络服务器和源文件等进行交互。
So that part is I would say more of the same model. I mean, it's not exactly the same. Like there's many new technologies. We have many people working on things like GPU profiling that are very different from what you would do with the CPU. But I would say you can think of it as very similar, just another iteration of what we've been doing the whole time. There's a second thing which is a bit different, which is understanding the models themselves and how they behave. And that one is quite nice, completely open-ended. Mostly because the models are changing very fast. But also the applications around those models are still fairly early in their life cycle.
这一部分,我认为可以说与之前的模型大同小异。虽然不是完全一样,因为有很多新技术。我们有很多人在研究类似GPU性能分析这样的工作,这与用CPU时的做法非常不同。但总的来说,你可以把它看作我们一直在做的事情的又一次迭代。第二个方面有一点不同在于对模型本身及其行为的理解。这部分相当不错,是完全开放的。主要原因是模型变化特别快,而围绕这些模型的应用程序还处于生命周期的早期阶段。
I think, you know, we, there's a few things that we understand well. So we understand what an image model looks like, you know, and we understand how to build a chatbot and those form factors are sort of there. But for the rest, we're still looking for the right form factors and the models themselves, as I said, keep changing underneath that. So I think it's going to take maybe a few years for that category of observing the models themselves to fully flesh out in terms of what the use cases are, the needs and where they go. And you just announced something right that Dash, which is a UN, your conference, an LLM observability product that does some of this? Yes, yes. We named it so it's easy to pronounce LLM observability, try to say it four times in a row. I had to practice hard for the keynote for that.
我觉得我们对一些事情理解得比较透彻。比如我们了解图像模型的样子,也知道如何构建聊天机器人,这些形式我们都比较熟悉。但是对于其他东西,我们仍在寻找合适的形式,而且模型本身也在不断变化。所以,我认为可能需要几年时间才能完全弄清楚这些模型在不同用例和需求下的应用方向。你刚才提到了一些事情,对吧?就是那个Dash,一个在联合国举行的会议,推出了一个LLM可观测性产品,对吧?是的,我们给它取了个容易发音的名字,叫做LLM可观测性,试着连续说四遍。这次大会的主题发言我可是做了不少练习。
And from what I read about LLM observability, the, so you do indeed like a personal performance, but very much to the point that you were just making, you also evaluate functional quality. So the quality of the results, which is new territory for data dark, right? Yes. The functionally observing software was easier when everything was deterministic. Now that the software changes over time and it's, humans might actually disagree on whether it behaves properly or not. I think it's becomes a much harder task. But again, that means there's more value in understanding that and bridging the gap between the humans and the, what the application is actually doing. And we're still fairly early there.
根据我所读到的关于LLM可观察性的内容,可以看出,确实像个人绩效一样进行评估,但正如你刚才提到的一样,也需要评价功能质量。评估结果的质量对于数据黑洞来说是一个新领域,对吧?是的。当一切都是确定性的时候,功能性观察软件要容易得多。现在,软件会随着时间改变,人们可能对其表现是否正确产生分歧。我认为这变成了一项更艰难的任务。但与此同时,这也意味着理解它和弥合人类与应用程序实际行为之间的差距变得更有价值。我们在这方面还处于相对早期的阶段。
Like we have a product that is out, we have real customers that use it with production applications, which is great. I mean, you know, you're a good, was not the case. Like everybody was talking about charge GPT, but other applications in the world were very few and far between. Now we start seeing that happen, but I expect that feel to change quite a bit in the near future. So, but it is out and you have customers in the, in the sort of evolution of a new product that you described, it says the design partner stage where people are sort of.
我们有一款已经发布的产品,并且有真实客户在生产环境中使用,这非常棒。我的意思是,过去并不是这样的情况。虽然大家都在谈论聊天生成模型(如ChatGPT),但实际上其他应用却很少见。现在我们开始看到这种情况在发生变化,我预计这种感觉会在不久的将来有很大的改变。总之,我们的产品已经推出,并且你在产品的进化阶段中就有客户使用,这就是你所说的设计合作伙伴阶段,人们在这个阶段......
But I would say it's different from other products in that most other products go after categories that are fairly mature, where the use cases on the customer side are fairly clear. I would see this one is still in motion. Like it's very possible that two years from now, the applications look very different. The applications built on top of LAMS or models in general look very different. So that's one theme of like the impact on the business and the opportunities and it's a, yeah, so it's like one more way that I guess just like that's unbelievable business, which is that like anything that, any new thing that pops up fundamentally is just another thing to monitor.
我会说,这款产品与其他产品不同,因为大多数其他产品都面向那些相对成熟的类别,这些类别的客户使用案例相对明确。我认为这款产品仍在发展中。比如,两年后它的应用可能会非常不同。基于LAMS或模型的应用可能会大不一样。这是一个对业务影响和机会的主题,也是令人难以置信的一点,即任何新出现的事物都会成为需要监控的新事物。
Yeah. And look, the strength of the business is that it usually doesn't make sense to look at one aspect in isolation. You are not going to manage your AI separately from your databases separately, from your network separately, from your security separately, from everything makes more sense when it is managed together and when we can assemble the full picture for you. So that's what we focus on. The last aspect on the impact on the owner business is obviously there's a lot we can do with AI ourselves on the back of it, which is we have all these data, all these use cases.
好的,公司的优势在于,我们通常不应该单独看待某一个方面。不能只是单独管理人工智能、数据库、网络或安全等,它们在一起统一管理时更有意义,这样我们才能为你提供完整的解决方案。所以这是我们的工作重点。对于公司本身的影响,显然有很多事情我们可以通过人工智能来完成,因为我们拥有大量数据和实际应用场景。
What can we do to automate that for you? And I would say this is an area that was, for the longest time in the history of the company, we've been careful about using the word AI. We thought there were bullshit words, mostly people would say AI, but in practice, this was statistics or even less chartably just addition, sub-sarcasm, voltifications. I mean, obviously now it's different. We definitely can do a lot more with it. But the promise here is since we are of the cleanest data that you use today to get people up at night, so when it's not clean, it gets fixed pretty quickly. And we are in the middle of the use cases, like the transaction use cases of, hey, I need to fix something and verify that it works. We are in the ideal insertion point to automate a lot of that. I vividly remember that part of the conversation from a few years ago when we did that chat where you were precisely using the example of waking somebody up and false positive versus false negative. And yeah, you did seem careful about machine learning at the time. Having said that so fast forward to today, you were saying this shortly after launching watchdog, I believe, which is the AI product I'll let you describe it better than I can. But there was a little bit that you were doing. And so generally the AI has increased, not just the scope or you can do, but also the level of confidence you have in terms of not working people up at night. Yes, but I think most importantly, we don't have to lead too hard with it.
我们可以怎么做来为您实现自动化?我想说,在公司历史上,我们一直对使用“人工智能”这个词很谨慎。过去,我们认为“人工智能”这个词有些夸大,大多数情况下只是统计学,甚至更简单的加减法。不过,现在情况不同了,我们确实可以做得更多。这个承诺在于,我们拥有非常干净的数据,这些数据可用于快速修复问题。我们正处在许多使用场景的核心位置,比如交易场景中,需要修复和验证的任务,我们是自动化这些任务的理想选择点。我清楚地记得几年前我们聊天时关于叫醒某人和误报与漏报的那部分讨论。在那时,你确实对机器学习很谨慎。不过,快进到今天,你在推出AI产品Watchdog后不久就提到了。你比我更能详细描述这个产品,但是一般来说,人工智能不仅扩大了我们可以做的事情范围,也提高了我们在夜晚不打扰他人的信心。最重要的是,我们不需要过于依赖这个词。
The problem with AI in general is if you start solely with the promise of automating with AI, you sort of on the hook to doing it all the time. And the general case for what we do is not something that you can solve with AI. I think right now we'd be lucky to solve 2%, 3%, 5% of the cases with AI. Maybe you know you're going to be 20%, maybe, but it's going to be gradual. I think if you try and insert yourself saying, I'm going to automate it for you, the incentive will be to try and do too much, break the confidence with a user, and then working out in the end, which has been the story of AI business, in systems management for the past 20 years, basically, that cycle has repeated again and again and again.
人工智能的一个普遍问题是,如果你的初衷仅仅是用AI来实现自动化,那么你就好像被迫一直去做。但我们所面对的一般性问题并不是单靠AI就可以解决的。我认为,目前我们可能只能用AI解决2%、3%、5%的问题。或许在未来,你能达到20%,但这将是一个渐进的过程。我觉得如果你一开始就说:"我来帮你实现自动化",那么往往会尝试做得太多,导致用户失去信心,然后再修复问题。这其实是过去20年里AI在系统管理领域的商业模式一直在重复的故事。
I think our strength comes from the fact that we can pick and choose. We can say, hey, for observability, we're here for security, we're here to make sure you can solve your issues, and little by little, we'll do more of it for you, up to the point where maybe you have only one person of the issues yourself. Maybe it makes sense to just double click on what AI means for the kind of data that you're dealing with, because we're not talking about creating avatars or voices or marketing copy, we're talking about detecting anomalies across mostly structured number, information everywhere, which to the point of having that watchdog product back in 2018 or 19 or whenever that was, that's very much what is now known incorrectly, but as machine learning versus generally AI.
我认为我们的优势在于我们可以自主选择。我们可以说,嘿,对于可观测性,我们在这里;对于安全,我们在这里;我们在这里确保您可以解决问题,逐渐地,我们会为您做得越来越多,直到您几乎只需应对极少的问题。也许应该仔细考虑一下AI在处理您数据时的意义,因为我们不是在说创造虚拟形象、声音或营销文案,而是在谈论在主要是结构化的数字信息中检测异常。这与2018或2019年我们推出的看门狗产品有关,这种技术现在通常被错误地称为机器学习,而不是通常的人工智能。
So what is the mix of different models you use? Yeah, so the first generation of products we've built there, we're built on statistical methods and traditional machine learning, which means the best way to describe it is not transformers. And that's worked really well, like this fit for purpose works really, really well. I think with the new generation of models that we've seen come up, what's relevant to us is first of all the language models themselves are very relevant, because now we also can access not just the numerical data we have, but we can access the documentations or customers everything but their systems, what they're saying on Slack and email, what's going on in their incidents. And these together, like the semantic meanings of the various things they even put into the events, the logs and things like that. So that's very interesting and opens up more things there. There's more we can do maybe on the reasoning side, I would say even with the latest releases from OpenAI, it's still fairly early in terms of the quality of the models and what can be done there.
你用的不同模型组合是什么?是这样的,我们最初开发的产品基于统计方法和传统的机器学习,也就是说,它们不是基于transformers模型的。这种方法非常有效,特别适合我们的需求。我认为,在我们看到的新一代模型中,首先对我们有意义的是语言模型本身。这些模型不仅让我们访问数值数据,还可以获取文档、客户系统中的内容,以及他们在Slack和电子邮件上说的内容,以及他们面临的事件。这些信息的语义意义结合在一起,使我们在那里可以做更多的事情。在推理方面,我们可能有更多的潜力。即使是OpenAI的最新发布,在模型质量和可实现的功能方面仍然处于比较早期的阶段。
And then what we're seeing also is how we can apply these amazingly scaling transformers technology to what we used to do with traditional machine learning, which is time series, events and even before that like statistical modeling. And speaking of which another thing that you announced, which I believe is not in production yet, is you've just announced your own foundation model, which is called Toto. What is the idea behind doing a foundation model for time series data? Well, so the idea is now that this technology has been proven to work really, really well, what can we do with our own data? Can we actually improve forecasting for own time series, for observability time series, and maybe also for other kinds of time series? So Toto was our first attempt. And what's really interesting is that even though it's our first attempt, the first time we incorporate transformers in what we do. And it's also not a gigantic model. Like it's very far from like it's not billions of parameters, like it's much more than that.
我们现在看到的是如何将具有惊人扩展能力的Transformer技术应用到我们过去用传统机器学习进行的工作中,比如时间序列、事件分析,甚至再往前追溯到统计建模。说到这点,你们最近宣布了一个我相信还未投入生产的项目,就是你们推出了自己的基础模型,名为Toto。为什么要为时间序列数据开发一个基础模型呢?
其背后的想法是,既然这项技术已经被证明效果非常出色,我们能否利用自己的数据来改进时间序列的预测,比如观察数据的时间序列,甚至其他类型的时间序列。因此,Toto是我们首次尝试。值得注意的是,即使这是我们的首次尝试,并首次在工作中引入了Transformers,它也不是一个巨大的模型,它远远没有达到数十亿参数的规模。
This model from the one with state of the art, like it beats all the other models. Of course, on the observability data, we have special benchmarks from that, but also for other things like weather data, which was very surprising to us. The reason for that is that we've just got amazing data for it. Like we've got, of course, tons of time series data, but we also have really strong metadata to understand the quality of the data. So going back to what I was saying earlier, we know which time series are working people up at night. We know which time series are being looked at and hoof on which dashboard, which give us really strong signals in terms of quality. So the plan now is to double down on that. So there's more we can do to make that model bigger, so more data, larger model size. And there's more we can do also to incorporate more types of data into it. So, I mean, multimodal data, though, you know, it's not in the sense of images and sound. It's in the sense of mixing text data and time series data in the same models, basically.
这款模型在某些方面达到了顶尖水平,甚至超过了其他所有模型。当然,这主要是在可观测数据的基准测试中取得的,但在其他领域,比如天气数据方面也有出乎意料的表现。其原因在于我们拥有出色的数据:不仅有大量的时间序列数据,还有强大的元数据来帮助理解数据质量。就像我之前提到的,我们知道哪些时间序列会让人夜不能寐,也知道哪些时间序列会被查看并在仪表盘上优先显示,这为我们提供了非常有力的数据质量信号。因此,我们计划继续深耕这一领域。我们可以做得更多,比如增加数据量,扩大模型规模。我们还可以整合更多种类的数据,主要是结合文本数据和时间序列数据的多模态模型,而不是图像和声音数据。
All right. So we talked about LLM observability. We talked about Toto. We sort of touched on Watchdog, which again is a product from a few years ago that you've kept on improving. What does Watchdog do? So Watchdog does the anomaly detection. So basically, the idea is when you use us, you're going to generate millions or billions of time series and logs and things like that. And it's just impossible for humans to watch all of them and understand if something's going wrong. So the idea with Watchdog is it just watches them for you. And it tells you, okay, so that thing is deteriorating. You should pay attention. Or that thing is assigned that other issue is going to happen later on the world. Moving back to the conversation we had a few years ago, the most important when you do things like that is to not generate false positives.
好的。我们讨论了LLM可观察性,谈到了Toto,也简单提到了Watchdog。这款产品是几年前推出的,并且你们不断在改进。Watchdog做什么呢?它负责检测异常。基本上,当你使用我们产品时,你将生成数百万甚至数十亿的时间序列和日志等数据。这些数据量大到人类无法实时监控并判断是否出现问题。所以Watchdog的理念就是替你监控这些数据。它会提醒你,比如某个数据在恶化,需要你注意,或者某个情况预示着将来可能会出现其他问题。回到我们几年前的讨论,进行这样的监控时,最重要的是不要出现误报。
These systems tend to generate more false negatives than false positives. Basically, the system is not sure. It's probably not going to tell you anything, because if it tells you things are going wrong twice and you don't believe it, you're never going to look at it again. So I think as the technology improves, as the quality of the models and the forecasting and the detection improves, we can go from having something very interesting to say, in 20% of the cases to maybe all the time, which would be amazing. And then maybe the last AI product to cover, at least in the context of this conversation, Bits AI, what is up? So Bits AI was the first time we incorporated the LMs into our product. We did the thing that was pretty much everyone's first attempt at incorporating Ginii, which was, hey, we have all these data and all these things in our product. In addition to our UIs, let's add a chatbot to it.
这些系统往往产生的假阴性多于假阳性。基本上,这意味着系统不确定。它可能不会告诉你任何事情,因为如果它告诉你两次事情出了问题而你不相信它,你就不会再去关注它了。所以我认为,随着技术的进步,以及模型、预测和检测质量的提高,我们可以从在20%的情况下有重要发现,到几乎每次都有发现,那将是非常了不起的。最后,也许在这次对话中会提到的最后一个AI产品是Bits AI,它是什么呢?Bits AI是我们第一次把大型语言模型应用到产品中。我们做的事情和几乎所有人第一次尝试集成Ginii时的做法一样,也就是说,嘿,我们的产品中有这么多数据和信息,除了用户界面之外,让我们再加一个聊天机器人。
So we can ask for anything, mix data from a responsible product and interact with it in text. Which we find is, and I think we've been through the same path as the most other companies that have done that, which is, it works great for some use cases, but you need to hang and hold the users a lot more. Users don't necessarily know exactly what to ask for and hold to ask for it, or what to go next in a once to ask a question. And we've all been there like we all tried the various bots, whether it's the Google one, the Microsoft one, and you start to try them, you force yourself to try them, and then you run out of ideas, so you don't come back. I think it's a very ironic considering it's also supposed to be easier, but in fact, it's harder. Yes, yes.
所以我们可以请求任何东西,将来自负责产品的数据混合,并以文本方式进行交互。我们发现,与大多数其他公司走过的路一样,这在某些用例中效果很好,但需要对用户进行更多的指导和帮助。用户不一定确切知道要询问什么,也不知道在询问一个问题后接下来该怎么做。我们都有过这样的经历,比如尝试各种聊天机器人,无论是谷歌的还是微软的。起初你强迫自己去尝试它们,但很快你就没有想法了,于是也就不再使用了。我认为这很讽刺,因为这些技术本应让事情变得更简单,但实际上却更复杂了。对,对。
So, but the next step for that really is, so that's great. I mean, there's still some advanced use cases where it's fantastic. People want to mix data and do things and that works great. However, in most cases with the models and the AI actually let us do is get ahead of the issues and the head of where the customer is arguing and help them get their faster. And so that's the next generation of that. We announced that at Dash. I'm going to fill your AI bingo card, but you know, you see the the the agentic workflows, you know, but the idea there being is the the machines that he is in its own loop and it's going to figure out what to do next and tell you about it. You don't have to ask. And so there's interesting ways for us to do that. Is that experimental? Is that working? Well, we have we actually it's experimental and working, you know, it's not something we've rolled out widely. But the idea there is say you get a page because something broke on your application. And by the time you get on on Slack, the body's there already and told you and he's telling you, this is what I looked into. I looked at check this piece of data, this piece of data. This is a notebook where I put my investigations. You can you can follow what I did there. I think this is probably that. My recommendation is to restart the service. You want to click here and restart it. Turn it off, sir. Oh, man. Yes, exactly. This is this is not this is really exciting.
这段话的意思可以翻译成中文如下:
所以,接下来的步骤确实很重要,这很棒。我的意思是,仍然有一些高级用例非常出色,人们希望混合数据并做一些事情,那很好用。然而,在大多数情况下,模型和AI确实让我们能够在问题发生之前采取行动,并在客户提出质疑之前帮助他们更快地解决问题。这就是下一代的功能。我们在Dash大会上宣布了这一点。我可能要填满你的AI术语表,你知道,所谓的代理工作流,其想法是机器在自己的循环中运行,它会弄清楚下一步该做什么并告诉你,你不需要问。而我们有一些有趣的方法来实现这一点。这样的技术是实验性的?是否可行?实际上,这既是实验性的也是可行的,但我们还没有广泛推行。比如说,如果你的应用程序出现问题,你收到一个页面提示,当你登录Slack时,助手已经在那里告诉你,我查看了这段数据,那段数据,并在笔记本中记录下我的调查。你可以跟随我做了什么。我认为问题可能出在这里。我的建议是重启服务。你可以点击这里重新启动它。关闭它,是的,没错。真的令人兴奋。
Like, you know, it really changes the interaction. Also, what she is what people do on Slack, you know, so when you when you when we ask a question about something, you can say, actually, I found the data for that. And this is what it is. Again, I think there we still we need to make sure it works well enough in enough of the cases because the models themselves are still imperfect enough that sometimes, you know, don't get exactly what you want. And also we need to make sure we find the right form factor for the interaction with the user, like what's not enough? What's too much? You know, you don't want to end up with Clippy. And the the risk right now is that a lot of the assistance we're seeing from the big companies are too close to Clippy. But for the young people who listen, Clippy was the the Microsoft word. Yes. Maybe to make sure that we at least touch upon it.
你知道,这真的改变了互动方式。而且,她就是人们在Slack上做的事情的典型例子。所以当我们提问某件事的时候,你可以说,其实我找到了相关数据,就是这个。不过,我认为我们仍需要确保在很多情况下这个工具足够好用,因为这些模型本身还存在很多不完美,有时候得不到你想要的结果。而且,我们还需要找到与用户互动的合适形式,比如什么是不够,什么是太多,你知道的,我们不想弄得到处都是Clippy。现在的风险是,我们从大公司看到的很多助手都太接近Clippy了。对于年轻人来说,Clippy是微软Word中的一个助手。我们至少要提到这一点。
The foundation for all of this, the reason why all this data in our able to leverage the data to fit it into the AI is because you have this unified real-time data platform, which, you know, sounds like a complete monster in terms of what does it do? Like trillions of data points per hour. How is it architected? Like, how does one build a platform that's able to do that kind of, you know, have that kind of performance? So first there's the way that is organized for that.
所有这些的基础,之所以我们能够利用这些数据并将其融入到人工智能中,是因为我们拥有一个统一的实时数据平台。这个平台听起来像是一个庞然大物,它能够每小时处理数万亿个数据点。它的架构是怎样的?如何构建一个能够展示这种性能的平台呢?首先,要谈谈它的组织方式。
You know, from day one, we have decided it would be a broad platform and it would load data from many different sources, more sources we didn't know at the time with different sizes, different velocities, different shapes. So we organize everything so that we could have we could hook together many different data stores under one one fairly flexible data mall. So that was the, I would say the the good idea in the beginning that helped us do that, even though it stood in the way of us getting into white community.
从第一天起,我们就决定打造一个广泛的平台,可以从许多不同来源加载数据。这些数据来源在当时我们并不完全清楚,有着不同的规模、速度和形式。因此,我们组织了一切,以便能够将许多不同的数据存储灵活地连接到一个数据中心。我会说,这一开始的好主意帮助我们实现了这个目标,尽管它阻碍了我们进入某个特定社区。
The after that, I think the secret has been we just keep rebuilding all of those modules all the time. Obviously we're not around the same data stores right now, you know, we which are getting billions of data points every second. Then you know, the things we had on week one, you know, what you were only going to just one database and there was extremely naive in terms of the, the Indian infrastructure.
之后,我认为我们的秘诀就是不断重建所有这些模块。显然,我们现在不再使用相同的数据存储,因为我们每秒都获得数十亿的数据点。你知道,第一周的时候,我们只连接了一个数据库,那时在基础设施方面非常简单。
And that's a you're right that it's a extremely high constraint word, especially since we have to keep that going, you know, 24 seven. And anytime we have a delay of a few seconds, it actually impacts our customers. So it's a the bar is fairly high in terms of we can do there. And the repercussions on the on the AI side is that the bar is fairly high also in terms of what we can incorporate on the AI side, what the cost of it can be to operate at this very large scale.
这确实是一个非常严格的限制,尤其是我们需要全天候不间断地运行。任何几秒钟的延迟都会对我们的客户产生影响。所以在这方面,我们的要求相当高。在人工智能方面,也是如此,我们可以加入的东西以及在如此大规模运行情况下的成本,同样都有很高的标准。
And also the latency of it can be in layman's terms that I get just like a gigantic cluster in the cloud that is like process cranks this whole thing and you have like connectors and feed data into it and API is to push data out of it is that that roughly the I mean, well, I mean, there's a there's a number of cues and data stores basically. And some of it is completely homegrown after generations of iterations.
这段话的大意是:“我们可以用通俗的语言来描述它的延迟现象。它就像是一个在云端的巨大集群,负责处理整个过程。你会有一些连接器把数据输入进去,还有API把数据输出出来。基本上有很多的队列和数据存储。其中有一部分是经过多代迭代后完全自主开发的。”
And some of it is still like open source on the shelf that works very well for us like we use a lot of Kafka, for example, of that works well, and we still use a lot of Kafka database wise, you know, we mostly built on and we still use in some areas like some standard databases like post-glasses and things like that. But we we obviously show them and scale them a lot.
其中一部分仍然是开源工具,对我们非常有用。例如,我们大量使用Kafka,这很适合我们的需求。在数据库方面,我们主要还是使用一些标准数据库,比如PostgreSQL等等。不过,我们在这些数据库的使用上进行了很多优化和扩展。
But a lot of the the core that I store like the time series, the log data, the even data, all of that is on completely custom data stores that we've built over time. We published actually a few series of articles on our on your event store, which we use for logs and traces, which is called Husky, you know, another dog name. And people can go on our blog and read about it like we share some of the technical details behind it. And you store all of this right? Like I think I read that there were some improvements around, you know, storing log data and that kind of stuff. But that's that you just store massive amount of historical data as well and be out of your customers.
我们存储了很多核心数据,比如时间序列、日志数据、事件数据等,这些数据都存储在我们逐步打造的完全自定义的数据存储中。我们实际上在博客上发布了一系列关于我们的事件存储的文章,我们称之为Husky,也就是另一种狗的名字,这是我们用于存储日志和跟踪的数据存储系统。人们可以在我们的博客上阅读这些文章,其中分享了一些技术细节。你也在存储这些数据,对吧?我记得有一些关于改进日志数据存储的讨论。不过,你确实存储了大量的历史数据,并且这些数据来自于你的客户。
Yeah. And we did some things there. I mean, look, the biggest challenge is observability is that any application can generate any any arbitrary large amount of logs. And so the data volumes grow much faster than our customers revenue, which is a problem. And so for to solve that, the few things to do, one is you create the right feedback loop. So people understand what they would they need and what they don't need in terms of data produced by the application. And when they send too much, you can fix it.
是的,我们在那方面做了一些工作。最大的挑战是可观测性,即任何应用程序都能生成任意数量庞大的日志。因此,数据量的增长速度远远超过客户的收入增长,这就是个问题。为了解决这个问题,我们可以做以下几件事情:首先,建立一个合适的反馈机制,让人们了解在应用程序生成的数据中哪些是需要的,哪些是不需要的。当他们发送过多数据时,可以进行调整。
But the other one is also you just to be need to be more and more efficient in terms of how you can send more data and store more data. And and it costs less. One thing we've done over the past few years is we decoupled the storage from the compute. So it allows us to store storage was much cheaper. They are at different, different basically, differently from the the compute, which is a lot more expensive. And you have about half of the team that works on that platform.
但另一个方面是,你需要越来越高效地传输和存储数据,并且降低成本。过去几年里,我们做的一件事就是将存储和计算分离开来。这使我们能够以更低的成本进行存储,因为存储的费用比计算便宜得多。大约有一半的团队成员在负责这个平台的工作。
So yes, the breakdown is roughly half of our engineering team is on the platform and half is on the is assigned to specific products. And for the AI stuff, did you have to build like a mini lab with some AI PhDs type? We so we don't have a lab in general. We're a little bit careful with with labs. I think it's a system expectation. But we so we and we've been through the same iterations as most companies, which is you centralized a little bit too much, then you decentralized a little bit too much, then you recent for a little bit.
好的,大概情况是这样的:我们的工程团队大约一半在负责平台,另一半专注于特定的产品。对于AI这方面的工作,你们是不是需要建立一个小型实验室,招募一些AI博士之类的人才呢?其实我们并没有专门的实验室,我们对实验室设置保持谨慎态度。我认为这是一种系统预期。我们经历了和大多数公司一样的过程,就是一开始有点过于集中,然后又有点过于分散,接着又适度地重新集中。
So you sort of going between those two to make sure you you get the right amount of financial, common stuff. And at the same time, the right amount of work is directly applicable to the real problems of real products and real customers. But we've been building that. I think the main difference in terms of the way we invest in we build the products for compared to the others is that because that part of the market is moving so fast, it's a little bit more speculative often, like you have to start building and experiment before you know exactly what it is you need. Just because one customer doesn't know yet. But two, you also need to learn as you go. So I think we place the dial a little bit differently there than we might in some other parts of the company.
你需要在两者之间找到平衡,确保在财务和共通事务上做到合理,同时确保所做的工作能够直接应用于真实产品和客户面临的实际问题。我们一直在努力构建这一点。我认为,与其他公司相比,我们在投资和产品研发方式上的主要区别在于,这个市场的发展非常迅速,因此经常带有一定的投机性。你需要在明确需求之前就开始建造和实验,因为客户有时还不了解自己的需求。而且,你也需要在这个过程中不断学习。因此,我认为我们在这方面的调整方式可能和公司的其他部分有些不同。
Incredible. Well, look, this has been a wonderful conversation, maybe too close and going in a completely different direction, but since you mentioned, you know, PG. And by the way, it's it's it's wonderful to hear that even YC makes terrible mistakes and terrible passes from from time to time that they just don't have like, you know, mega hits. But since you mentioned PG a couple of times and I heard you say either in our conversations or in other things I listened to that you at some point, you were a little bit of a control freak. What's your take on founder mode? Look, I think one it's a most of the would say in there is a value of your slack. You mean you you don't just, you know, tell people hire people and let them go and do whatever they want. Like you actually actually have to care about the details. Yes, you do. Like I think that parts value of use.
难以置信。好吧,你看,这一直是一场非常精彩的对话,也许有些太接近并且走向了完全不同的方向。不过,既然你提到了PG。顺便说一句,很高兴听到即使是YC也会时不时犯下重大错误,并不总是能够取得巨大的成功。但是,既然你几次提到了PG,我听你说过,无论是在我们的对话中,还是在我听到的其他事情中,你曾经有点控制狂。你对创业者模式有什么看法呢?我认为大多数人会说,保持一些控制是有价值的。我的意思是,你不能仅仅告诉人们去招聘然后就让他们随意行动。你确实需要关心细节。是的,这确实很重要,我认为这部分是有价值的。
My worry about the founder mode and everything that gets pulled down to a very short piece like that is that I think it's going to be using that using all sorts of different ways because there's so much context that goes into every single world in there. Like when you say manage someone, what does it mean? Like it's going to mean very different things for the very, very different people or details when you mean very different things for very different people. So I think it's a as is that my worry is that it's going to do more harm than good in terms of the impact on the on the ecosystem. I've already been on the receiving end of a few people quoting quoting founder mode to disagree with things that we're doing. I was doing so.
我对“创始人模式”和把所有内容压缩到一小段的方式感到担忧,因为我觉得它可能会被用在各种不同的场合。每个字词中都有大量背景信息。例如,当你说“管理某人”时,这是什么意思?对不同的人而言,它可能意味着非常不同的东西。因此,我担心这可能对生态系统的影响弊大于利。我已经遇到过有些人用“创始人模式”来反对我们正在做的事情。
We'll see where it goes. But look, I point short of it is yes, of course you have to care about the details. I think that's where you build a company and I think that's a there's no way to to remain relevant unless you do that. OK, amazing. Thank you so much for doing this. Thank you.
我们拭目以待吧。不过,总的来说,答案是肯定的,当然你得关注细节。我认为这是建立公司的基础,我觉得如果不这么做,就无法保持竞争力。好的,非常感谢你来参与。谢谢。